Predicting Time to Diagnosis for the WiDS Datathon #2
Posted by Tanya Kuruvilla,
Introduction
- Importing a Tabular Dataset
- Preprocessing the Data
- Exploring Tabular Data
- Choosing and Creating Features
- Training a Machine Learning Model
- Making New Predictions and Exporting Submissions
Import Data
patient_id | patient_race | payer_type | patient_state | patient_zip3 | Region | Division | patient_age | patient_gender | bmi | breast_cancer_diagnosis_code | breast_cancer_diagnosis_desc | metastatic_cancer_diagnosis_code | metastatic_first_novel_treatment | metastatic_first_novel_treatment_type | population | density | age_median | age_under_10 | age_10_to_19 | age_20s | age_30s | age_40s | age_50s | age_60s | age_70s | age_over_80 | male | female | married | |
---|---|---|---|---|---|---|---|---|---|---|---|---|---|---|---|---|---|---|---|---|---|---|---|---|---|---|---|---|---|---|
1 | 268700 | ” | ‘COMMERCIAL’ | ‘AR’ | 724 | ‘South’ | ‘West South Central’ | 39 | ‘F’ | NaN | ‘C50912’ | ‘Malignant neoplasm of unspecified site of left female breast’ | ‘C773’ | NaN | NaN | 3.9249e+03 | 82.6283 | 42.5750 | 11.6050 | 13.0317 | 10.8667 | 11.8017 | 12.2917 | 13.2167 | 13.4717 | 10.0717 | 3.6350 | 51.4317 | 48.5683 | 51.0483 |
2 | 484983 | ‘White’ | ” | ‘IL’ | 629 | ‘Midwest’ | ‘East North Central’ | 55 | ‘F’ | 35.3600 | ‘C50412’ | ‘Malig neoplasm of upper-outer quadrant of left female breast’ | ‘C773’ | NaN | NaN | 2.7454e+03 | 51.7936 | 43.5351 | 11.2247 | 12.1922 | 11.4467 | 11.0065 | 11.3545 | 14.3922 | 14.1507 | 9.1727 | 5.0506 | 49.3234 | 50.6766 | 49.4753 |
3 | 277055 | ” | ‘COMMERCIAL’ | ‘CA’ | 925 | ‘West’ | ‘Pacific’ | 59 | ‘F’ | NaN | ‘1749’ | ‘Malignant neoplasm of breast (female), unspecified’ | ‘C773’ | NaN | NaN | 3.8343e+04 | 700.3375 | 36.2795 | 13.2667 | 15.6641 | 13.4949 | 13.4538 | 12,4000 | 11.5846 | 10.4667 | 6.3769 | 3.2846 | 49.9897 | 50.0103 | 48.8077 |
4 | 320055 | ‘Hispanic’ | ‘MEDICAID’ | ‘CA’ | 900 | ‘West’ | ‘Pacific’ | 59 | ‘F’ | NaN | ‘C50911’ | ‘Malignant neoplasm of unsp site of right female breast’ | ‘C773’ | NaN | NaN | 3.6054e+04 | 5.2943e+03 | 36.6538 | 9.7615 | 11.2677 | 17.2339 | 17.4415 | 13.0908 | 12.3046 | 9.4077 | 5.6738 | 3.8246 | 50.5108 | 49.4892 | 33.4785 |
5 | 190386 | ” | ‘COMMERCIAL’ | ‘CA’ | 934 | ‘West’ | ‘Pacific’ | 71 | ‘F’ | NaN | ‘1748’ | ‘Malignant neoplasm of other specified sites of female breast’ | ‘C7951’ | NaN | NaN | 1.3700e+04 | 400.4763 | 41.7816 | 10.0316 | 16.4342 | 12.9710 | 11.2921 | 10.0868 | 11.5605 | 13.2790 | 8.7842 | 5.5316 | 51.9895 | 48.0132 | 48.2079 |
6 | 559027 | ” | ‘COMMERCIAL’ | ‘IN’ | 461 | ‘Midwest’ | ‘East North Central’ | 63 | ‘F’ | NaN | ‘1749’ | ‘Malignant neoplasm of breast (female), unspecified’ | ‘C786’ | NaN | NaN | 9.3229e+03 | 274.7371 | 40.1237 | 12.2300 | 13.8800 | 11.5317 | 11.9350 | 12.5517 | 13.9117 | 13.0467 | 7 | 3.9067 | 50.9817 | 49.0183 | 57.1617 |
7 | 293747 | ‘White’ | ‘MEDICARE ADVANTAGE’ | ‘OH’ | 448 | ‘Midwest’ | ‘East North Central’ | 57 | ‘F’ | 33.1000 | ‘C50412’ | ‘Malig neoplasm of upper-outer quadrant of left female breast’ | ‘C799’ | NaN | NaN | 5.8906e+03 | 122.3929 | 42.4536 | 12.4286 | 13.1893 | 10.8089 | 10.7321 | 13.0411 | 13.2786 | 14.2804 | 7.5732 | 4.6786 | 49.9107 | 50.0893 | 55.8696 |
8 | 517596 | ‘White’ | ‘COMMERCIAL’ | ‘DE’ | 198 | ‘South’ | ‘South Atlantic’ | 56 | ‘F’ | 31.0500 | ‘C50411’ | ‘Malig neoplm of upper-outer quadrant of right female breast’ | ‘C792’ | NaN | NaN | 2.2036e+04 | 1.4505e+03 | 41.6300 | 11.0300 | 11.9800 | 12.1100 | 13.6900 | 11.6600 | 13.9500 | 12.9700 | 7.6900 | 4.9700 | 47.8200 | 52.1800 | 42.0300 |
9 | 533188 | ” | ‘COMMERCIAL’ | ‘LA’ | 706 | ‘South’ | ‘West South Central’ | 65 | ‘F’ | NaN | ‘C50212’ | ‘Malig neoplasm of upper-inner quadrant of left female breast’ | ‘C773’ | NaN | NaN | 7.2198e+03 | 531.0590 | 39.5421 | 12.4474 | 14.7868 | 11.0026 | 12.5368 | 11.6868 | 14.6947 | 12.4789 | 5.8816 | 4.4947 | 50.5500 | 49.4500 | 49.0737 |
10 | 639484 | ‘White’ | ‘COMMERCIAL’ | ‘CA’ | 922 | ‘West’ | ‘Pacific’ | 60 | ‘F’ | NaN | ‘C50912’ | ‘Malignant neoplasm of unspecified site of left female breast’ | ‘C773’ | NaN | NaN | 1.6550e+04 | 245.0979 | 44.2326 | 9.8872 | 10.4149 | 13.6723 | 11.3894 | 9.1447 | 15.5638 | 14.6277 | 10.1106 | 5.2298 | 54.2000 | 45.8000 | 46.5192 |
11 | 366431 | ‘Black’ | ‘MEDICARE ADVANTAGE’ | ‘PA’ | 191 | ‘Northeast’ | ‘Middle Atlantic’ | 71 | ‘F’ | NaN | ‘C50911’ | ‘Malignant neoplasm of unsp site of right female breast’ | ‘C7989’ | NaN | NaN | 3.1948e+04 | 5.5122e+03 | 35.7191 | 10.8532 | 10.9511 | 18.1596 | 17.3489 | 11.6468 | 11.0979 | 10.6425 | 5.9426 | 3.3511 | 48.3085 | 51.6915 | 32.4915 |
12 | 793091 | ‘White’ | ‘MEDICARE ADVANTAGE’ | ‘OH’ | 453 | ‘Midwest’ | ‘East North Central’ | 73 | ‘F’ | 23.6100 | ‘C50811’ | ‘Malignant neoplasm of ovrlp sites of right female breast’ | ‘C773’ | NaN | NaN | 6.4682e+03 | 196.6312 | 40.5818 | 12.2182 | 14.1455 | 12.2714 | 11.5338 | 12.0546 | 13.7792 | 12.8234 | 7.2948 | 3.8766 | 50.0740 | 49.9260 | 56.5662 |
13 | 834862 | ‘White’ | ‘MEDICARE ADVANTAGE’ | ‘MN’ | 481 | ‘Midwest’ | ‘West North Central’ | 47 | ‘F’ | NaN | ‘1749’ | ‘Malignant neoplasm of breast (female), unspecified’ | ‘C773’ | NaN | NaN | 1.2190e+04 | 249.1628 | 40.7686 | 12.8465 | 14.0198 | 10.0698 | 12.7035 | 12.9919 | 14.9977 | 12.1826 | 6.5198 | 3.6779 | 50.9942 | 49.0058 | 58.1977 |
14 | 834862 | ‘White’ | ‘COMMERCIAL’ | ‘MI’ | 481 | ‘Midwest’ | ‘East North Central’ | 47 | ‘F’ | 26 | ‘1749’ | ‘Malignant neoplasm of breast (female), unspecified’ | ‘C773’ | NaN | NaN | 2.3266+04 | 743.5571 | 41.4729 | 10.9443 | 13.5914 | 12.6671 | 11.6100 | 12.1371 | 14.6457 | 12.7271 | 7.9286 | 3.7514 | 49.4800 | 50.5200 | 50.2657 |
⋮ |
Variables:
patient_id: 13173×1 double
Properties:
Description: patient_id
Values:
Min 1.0004e+05
Median 5.5577e+05
Max 9.9998e+05
patient_race: 13173×1 cell array of character vectors
Properties:
Description: patient_race
payer_type: 13173×1 cell array of character vectors
Properties:
Description: payer_type
patient_state: 13173×1 cell array of character vectors
Properties:
Description: patient_state
patient_zip3: 13173×1 double
Properties:
Description: patient_zip3
Values:
Min 100
Median 557
Max 995
Region: 13173×1 cell array of character vectors
Properties:
Description: Region
Division: 13173×1 cell array of character vectors
Properties:
Description: Division
patient_age: 13173×1 double
Properties:
Description: patient_age
Values:
Min 18
Median 59
Max 91
patient_gender: 13173×1 cell array of character vectors
Properties:
Description: patient_gender
bmi: 13173×1 double
Properties:
Description: bmi
Values:
Min 15
Median 28.58
Max 97
NumMissing 9071
breast_cancer_diagnosis_code: 13173×1 cell array of character vectors
Properties:
Description: breast_cancer_diagnosis_code
breast_cancer_diagnosis_desc: 13173×1 cell array of character vectors
Properties:
Description: breast_cancer_diagnosis_desc
metastatic_cancer_diagnosis_code: 13173×1 cell array of character vectors
Properties:
Description: metastatic_cancer_diagnosis_code
metastatic_first_novel_treatment: 13173×1 double
Properties:
Description: metastatic_first_novel_treatment
Values:
Min NaN
Median NaN
Max NaN
NumMissing 13173
metastatic_first_novel_treatment_type: 13173×1 double
Properties:
Description: metastatic_first_novel_treatment_type
Values:
Min NaN
Median NaN
Max NaN
NumMissing 13173
population: 13173×1 double
Properties:
Description: population
Values:
Min 635.55
Median 18953
Max 71374
density: 13173×1 double
Properties:
Description: density
Values:
Min 0.91667
Median 700.34
Max 29852
age_median: 13173×1 double
Properties:
Description: age_median
Values:
Min 20.6
Median 40.639
Max 54.57
age_under_10: 13173×1 double
Properties:
Description: age_under_10
Values:
Min 0
Median 11.004
Max 17.675
age_10_to_19: 13173×1 double
Properties:
Description: age_10_to_19
Values:
Min 6.3143
Median 12.898
Max 35.3
age_20s: 13173×1 double
Properties:
Description: age_20s
Values:
Min 5.925
Median 12.532
Max 62.1
age_30s: 13173×1 double
Properties:
Description: age_30s
Values:
Min 1.5
Median 12.404
Max 25.471
age_40s: 13173×1 double
Properties:
Description: age_40s
Values:
Min 0.8
Median 12.124
Max 17.82
age_50s: 13173×1 double
Properties:
Description: age_50s
Values:
Min 0
Median 13.57
Max 21.661
age_60s: 13173×1 double
Properties:
Description: age_60s
Values:
Min 0.2
Median 12.518
Max 24.51
age_70s: 13173×1 double
Properties:
Description: age_70s
Values:
Min 0
Median 7.325
Max 19
age_over_80: 13173×1 double
Properties:
Description: age_over_80
Values:
Min 0
Median 3.8246
Max 18.825
male: 13173×1 double
Properties:
Description: male
Values:
Min 39.725
Median 49.976
Max 61.6
female: 13173×1 double
Properties:
Description: female
Values:
Min 38.4
Median 50.024
Max 60.275
married: 13173×1 double
Properties:
Description: married
Values:
Min 0.9
Median 49.434
Max 66.903
divorced: 13173×1 double
Properties:
Description: divorced
Values:
Min 0.2
Median 12.717
Max 21.033
never_married: 13173×1 double
Properties:
Description: never_married
Values:
Min 13.44
Median 32.011
Max 98.9
widowed: 13173×1 double
Properties:
Description: widowed
Values:
Min 0
Median 5.5507
Max 20.65
family_size: 13173×1 double
Properties:
Description: family_size
Values:
Min 2.5504
Median 3.16
Max 4.1723
NumMissing 5
family_dual_income: 13173×1 double
Properties:
Description: family_dual_income
Values:
Min 19.312
Median 52.592
Max 65.635
NumMissing 5
income_household_median: 13173×1 double
Properties:
Description: income_household_median
Values:
Min 29222
Median 69730
Max 1.6412e+05
NumMissing 5
income_household_under_5: 13173×1 double
Properties:
Description: income_household_under_5
Values:
Min 0.75
Median 2.8848
Max 19.62
NumMissing 5
income_household_5_to_10: 13173×1 double
Properties:
Description: income_household_5_to_10
Values:
Min 0.36154
Median 2.1986
Max 11.872
NumMissing 5
income_household_10_to_15: 13173×1 double
Properties:
Description: income_household_10_to_15
Values:
Min 1.0154
Median 3.7875
Max 14.278
NumMissing 5
income_household_15_to_20: 13173×1 double
Properties:
Description: income_household_15_to_20
Values:
Min 1.0278
Median 3.7883
Max 12.4
NumMissing 5
income_household_20_to_25: 13173×1 double
Properties:
Description: income_household_20_to_25
Values:
Min 1.1
Median 4.0421
Max 14.35
NumMissing 5
income_household_25_to_35: 13173×1 double
Properties:
Description: income_household_25_to_35
Values:
Min 2.65
Median 8.4349
Max 26.55
NumMissing 5
income_household_35_to_50: 13173×1 double
Properties:
Description: income_household_35_to_50
Values:
Min 1.7
Median 11.833
Max 24.075
NumMissing 5
income_household_50_to_75: 13173×1 double
Properties:
Description: income_household_50_to_75
Values:
Min 4.95
Median 17.076
Max 27.13
NumMissing 5
income_household_75_to_100: 13173×1 double
Properties:
Description: income_household_75_to_100
Values:
Min 4.7333
Median 12.677
Max 24.8
NumMissing 5
income_household_100_to_150: 13173×1 double
Properties:
Description: income_household_100_to_150
Values:
Min 4.2889
Median 15.938
Max 27.477
NumMissing 5
income_household_150_over: 13173×1 double
Properties:
Description: income_household_150_over
Values:
Min 0.84
Median 14.655
Max 52.824
NumMissing 5
income_household_six_figure: 13173×1 double
Properties:
Description: income_household_six_figure
Values:
Min 5.6926
Median 30.523
Max 69.032
NumMissing 5
income_individual_median: 13173×1 double
Properties:
Description: income_individual_median
Values:
Min 4316
Median 35211
Max 88910
home_ownership: 13173×1 double
Properties:
Description: home_ownership
Values:
Min 15.85
Median 69.91
Max 90.367
NumMissing 5
housing_units: 13173×1 double
Properties:
Description: housing_units
Values:
Min 0
Median 6994.4
Max 25923
home_value: 13173×1 double
Properties:
Description: home_value
Values:
Min 60629
Median 2.4116e+05
Max 1.8531e+06
NumMissing 5
rent_median: 13173×1 double
Properties:
Description: rent_median
Values:
Min 448.4
Median 1155.4
Max 2965.2
NumMissing 5
rent_burden: 13173×1 double
Properties:
Description: rent_burden
Values:
Min 17.791
Median 30.829
Max 108.6
NumMissing 5
education_less_highschool: 13173×1 double
Properties:
Description: education_less_highschool
Values:
Min 0
Median 10.745
Max 34.325
education_highschool: 13173×1 double
Properties:
Description: education_highschool
Values:
Min 0
Median 27.484
Max 53.96
education_some_college: 13173×1 double
Properties:
Description: education_some_college
Values:
Min 7.2
Median 29.286
Max 50.133
education_bachelors: 13173×1 double
Properties:
Description: education_bachelors
Values:
Min 2.4657
Median 18.871
Max 41.7
education_graduate: 13173×1 double
Properties:
Description: education_graduate
Values:
Min 2.0941
Median 10.777
Max 51.84
education_college_or_above: 13173×1 double
Properties:
Description: education_college_or_above
Values:
Min 7.0488
Median 29.793
Max 77.817
education_stem_degree: 13173×1 double
Properties:
Description: education_stem_degree
Values:
Min 23.915
Median 42.99
Max 73
labor_force_participation: 13173×1 double
Properties:
Description: labor_force_participation
Values:
Min 30.7
Median 62.778
Max 78.67
unemployment_rate: 13173×1 double
Properties:
Description: unemployment_rate
Values:
Min 0.82308
Median 5.4857
Max 18.8
self_employed: 13173×1 double
Properties:
Description: self_employed
Values:
Min 2.263
Median 12.73
Max 25.538
NumMissing 5
farmer: 13173×1 double
Properties:
Description: farmer
Values:
Min 0
Median 0.45493
Max 25.267
NumMissing 5
race_white: 13173×1 double
Properties:
Description: race_white
Values:
Min 14.496
Median 70.904
Max 98.444
race_black: 13173×1 double
Properties:
Description: race_black
Values:
Min 0.08
Median 6.4103
Max 69.66
race_asian: 13173×1 double
Properties:
Description: race_asian
Values:
Min 0
Median 2.8214
Max 49.85
race_native: 13173×1 double
Properties:
Description: race_native
Values:
Min 0
Median 0.42759
Max 76.935
race_pacific: 13173×1 double
Properties:
Description: race_pacific
Values:
Min 0
Median 0.05
Max 14.758
race_other: 13173×1 double
Properties:
Description: race_other
Values:
Min 0.002564
Median 3.52
Max 33.189
race_multiple: 13173×1 double
Properties:
Description: race_multiple
Values:
Min 0.43333
Median 5.65
Max 26.43
hispanic: 13173×1 double
Properties:
Description: hispanic
Values:
Min 0.060714
Median 11.983
Max 91.005
disabled: 13173×1 double
Properties:
Description: disabled
Values:
Min 4.6
Median 12.955
Max 35.156
poverty: 13173×1 double
Properties:
Description: poverty
Values:
Min 3.4333
Median 12.209
Max 38.348
NumMissing 5
limited_english: 13173×1 double
Properties:
Description: limited_english
Values:
Min 0
Median 2.7472
Max 26.755
NumMissing 5
commute_time: 13173×1 double
Properties:
Description: commute_time
Values:
Min 12.461
Median 27.786
Max 48.02
health_uninsured: 13173×1 double
Properties:
Description: health_uninsured
Values:
Min 2.44
Median 7.3556
Max 27.566
veteran: 13173×1 double
Properties:
Description: veteran
Values:
Min 1.2
Median 6.9933
Max 25.2
AverageOfJan_13: 13173×1 double
Properties:
Description: Average of Jan-13
Values:
Min 6.7891
Median 35.412
Max 72.373
NumMissing 33
AverageOfFeb_13: 13173×1 double
Properties:
Description: Average of Feb-13
Values:
Min 8.9344
Median 36.71
Max 71.003
NumMissing 3
AverageOfMar_13: 13173×1 double
Properties:
Description: Average of Mar-13
Values:
Min 14.001
Median 40.585
Max 70.707
AverageOfApr_13: 13173×1 double
Properties:
Description: Average of Apr-13
Values:
Min 29.303
Median 53.65
Max 76.73
AverageOfMay_13: 13173×1 double
Properties:
Description: Average of May-13
Values:
Min 43.258
Median 63.891
Max 81.449
NumMissing 3
AverageOfJun_13: 13173×1 double
Properties:
Description: Average of Jun-13
Values:
Min 56.635
Median 71.18
Max 91.641
NumMissing 20
AverageOfJul_13: 13173×1 double
Properties:
Description: Average of Jul-13
Values:
Min 60.114
Median 74.462
Max 96.454
AverageOfAug_13: 13173×1 double
Properties:
Description: Average of Aug-13
Values:
Min 56.867
Median 72.511
Max 92.333
NumMissing 17
AverageOfSep_13: 13173×1 double
Properties:
Description: Average of Sep-13
Values:
Min 48.108
Median 68.27
Max 86.437
NumMissing 27
AverageOfOct_13: 13173×1 double
Properties:
Description: Average of Oct-13
Values:
Min 39.809
Median 57.171
Max 80.183
NumMissing 59
AverageOfNov_13: 13173×1 double
Properties:
Description: Average of Nov-13
Values:
Min 24.242
Median 43.371
Max 76.612
NumMissing 3
AverageOfDec_13: 13173×1 double
Properties:
Description: Average of Dec-13
Values:
Min -1.1231
Median 36.49
Max 74.47
NumMissing 3
AverageOfJan_14: 13173×1 double
Properties:
Description: Average of Jan-14
Values:
Min -2.863
Median 31.096
Max 70.775
NumMissing 4
AverageOfFeb_14: 13173×1 double
Properties:
Description: Average of Feb-14
Values:
Min 0.39012
Median 34.685
Max 73.245
NumMissing 9
AverageOfMar_14: 13173×1 double
Properties:
Description: Average of Mar-14
Values:
Min 13.962
Median 41.958
Max 72.13
NumMissing 29
AverageOfApr_14: 13173×1 double
Properties:
Description: Average of Apr-14
Values:
Min 32.845
Median 55.348
Max 76.205
NumMissing 180
AverageOfMay_14: 13173×1 double
Properties:
Description: Average of May-14
Values:
Min 46.646
Median 64.027
Max 80.57
AverageOfJun_14: 13173×1 double
Properties:
Description: Average of Jun-14
Values:
Min 51.611
Median 71.413
Max 90.224
NumMissing 152
AverageOfJul_14: 13173×1 double
Properties:
Description: Average of Jul-14
Values:
Min 57.604
Median 73.955
Max 95.528
AverageOfAug_14: 13173×1 double
Properties:
Description: Average of Aug-14
Values:
Min 56.561
Median 73.225
Max 90.17
AverageOfSep_14: 13173×1 double
Properties:
Description: Average of Sep-14
Values:
Min 42.48
Median 67.588
Max 87.833
AverageOfOct_14: 13173×1 double
Properties:
Description: Average of Oct-14
Values:
Min 34.796
Median 58.049
Max 82.105
AverageOfNov_14: 13173×1 double
Properties:
Description: Average of Nov-14
Values:
Min 19.001
Median 41.864
Max 74.565
NumMissing 24
AverageOfDec_14: 13173×1 double
Properties:
Description: Average of Dec-14
Values:
Min 15.782
Median 39.631
Max 72.174
AverageOfJan_15: 13173×1 double
Properties:
Description: Average of Jan-15
Values:
Min 9.6504
Median 34.297
Max 70.595
NumMissing 6
AverageOfFeb_15: 13173×1 double
Properties:
Description: Average of Feb-15
Values:
Min 0.39436
Median 33.389
Max 72.165
NumMissing 12
AverageOfMar_15: 13173×1 double
Properties:
Description: Average of Mar-15
Values:
Min 21.481
Median 45.209
Max 75.841
NumMissing 12
AverageOfApr_15: 13173×1 double
Properties:
Description: Average of Apr-15
Values:
Min 38.365
Median 55.409
Max 79.593
NumMissing 28
AverageOfMay_15: 13173×1 double
Properties:
Description: Average of May-15
Values:
Min 44.952
Median 64.963
Max 80.898
AverageOfJun_15: 13173×1 double
Properties:
Description: Average of Jun-15
Values:
Min 55.876
Median 71.144
Max 92.338
AverageOfJul_15: 13173×1 double
Properties:
Description: Average of Jul-15
Values:
Min 58.114
Median 74.724
Max 92.895
AverageOfAug_15: 13173×1 double
Properties:
Description: Average of Aug-15
Values:
Min 56.368
Median 74.452
Max 95.258
NumMissing 22
AverageOfSep_15: 13173×1 double
Properties:
Description: Average of Sep-15
Values:
Min 46.958
Median 71.177
Max 98.951
AverageOfOct_15: 13173×1 double
Properties:
Description: Average of Oct-15
Values:
Min 41.013
Median 57.607
Max 82.79
NumMissing 16
AverageOfNov_15: 13173×1 double
Properties:
Description: Average of Nov-15
Values:
Min 26.877
Median 48.956
Max 79.126
NumMissing 16
AverageOfDec_15: 13173×1 double
Properties:
Description: Average of Dec-15
Values:
Min 16.14
Median 46.322
Max 77.383
NumMissing 18
AverageOfJan_16: 13173×1 double
Properties:
Description: Average of Jan-16
Values:
Min 9.633
Median 33.117
Max 71.904
NumMissing 16
AverageOfFeb_16: 13173×1 double
Properties:
Description: Average of Feb-16
Values:
Min 14.552
Median 39.459
Max 77.696
NumMissing 16
AverageOfMar_16: 13173×1 double
Properties:
Description: Average of Mar-16
Values:
Min 29.155
Median 50.109
Max 74.822
AverageOfApr_16: 13173×1 double
Properties:
Description: Average of Apr-16
Values:
Min 35.264
Median 55.783
Max 76.571
AverageOfMay_16: 13173×1 double
Properties:
Description: Average of May-16
Values:
Min 45.325
Median 61.856
Max 79.608
NumMissing 19
AverageOfJun_16: 13173×1 double
Properties:
Description: Average of Jun-16
Values:
Min 55.897
Median 72.583
Max 94.287
AverageOfJul_16: 13173×1 double
Properties:
Description: Average of Jul-16
Values:
Min 60.402
Median 76.48
Max 95.633
NumMissing 16
AverageOfAug_16: 13173×1 double
Properties:
Description: Average of Aug-16
Values:
Min 58.124
Median 76.37
Max 96.091
AverageOfSep_16: 13173×1 double
Properties:
Description: Average of Sep-16
Values:
Min 50.671
Median 70.889
Max 85.494
AverageOfOct_16: 13173×1 double
Properties:
Description: Average of Oct-16
Values:
Min 37.083
Median 60.207
Max 79.631
AverageOfNov_16: 13173×1 double
Properties:
Description: Average of Nov-16
Values:
Min 25.945
Median 49.15
Max 75.547
NumMissing 3
AverageOfDec_16: 13173×1 double
Properties:
Description: Average of Dec-16
Values:
Min 9.8677
Median 36.823
Max 75.628
NumMissing 13
AverageOfJan_17: 13173×1 double
Properties:
Description: Average of Jan-17
Values:
Min 10.249
Median 37.942
Max 71.952
NumMissing 9
AverageOfFeb_17: 13173×1 double
Properties:
Description: Average of Feb-17
Values:
Min 17.485
Median 44.27
Max 72.402
AverageOfMar_17: 13173×1 double
Properties:
Description: Average of Mar-17
Values:
Min 20.439
Median 47.794
Max 73.785
AverageOfApr_17: 13173×1 double
Properties:
Description: Average of Apr-17
Values:
Min 38.856
Median 57.596
Max 80.696
AverageOfMay_17: 13173×1 double
Properties:
Description: Average of May-17
Values:
Min 46.06
Median 62.719
Max 82.129
AverageOfJun_17: 13173×1 double
Properties:
Description: Average of Jun-17
Values:
Min 53.403
Median 71.213
Max 92.757
NumMissing 1
AverageOfJul_17: 13173×1 double
Properties:
Description: Average of Jul-17
Values:
Min 58.14
Median 75.782
Max 106.73
NumMissing 31
AverageOfAug_17: 13173×1 double
Properties:
Description: Average of Aug-17
Values:
Min 55.428
Median 72.311
Max 94.479
AverageOfSep_17: 13173×1 double
Properties:
Description: Average of Sep-17
Values:
Min 49.352
Median 69.367
Max 85.72
NumMissing 10
AverageOfOct_17: 13173×1 double
Properties:
Description: Average of Oct-17
Values:
Min 38.41
Median 60.651
Max 79.556
NumMissing 21
AverageOfNov_17: 13173×1 double
Properties:
Description: Average of Nov-17
Values:
Min 23.168
Median 46.499
Max 75.306
NumMissing 5
AverageOfDec_17: 13173×1 double
Properties:
Description: Average of Dec-17
Values:
Min 8.609
Median 35.899
Max 71.741
AverageOfJan_18: 13173×1 double
Properties:
Description: Average of Jan-18
Values:
Min 5.9302
Median 33.93
Max 73.314
AverageOfFeb_18: 13173×1 double
Properties:
Description: Average of Feb-18
Values:
Min 4.1048
Median 42.023
Max 75.045
NumMissing 5
AverageOfMar_18: 13173×1 double
Properties:
Description: Average of Mar-18
Values:
Min 22.722
Median 43.237
Max 71.638
NumMissing 6
AverageOfApr_18: 13173×1 double
Properties:
Description: Average of Apr-18
Values:
Min 28.793
Median 50.292
Max 76.49
AverageOfMay_18: 13173×1 double
Properties:
Description: Average of May-18
Values:
Min 45.877
Median 66.117
Max 86.572
AverageOfJun_18: 13173×1 double
Properties:
Description: Average of Jun-18
Values:
Min 53.458
Median 71.642
Max 90.658
NumMissing 9
AverageOfJul_18: 13173×1 double
Properties:
Description: Average of Jul-18
Values:
Min 58.542
Median 76.647
Max 96.432
NumMissing 46
AverageOfAug_18: 13173×1 double
Properties:
Description: Average of Aug-18
Values:
Min 56.201
Median 76.079
Max 95.772
NumMissing 16
AverageOfSep_18: 13173×1 double
Properties:
Description: Average of Sep-18
Values:
Min 51.829
Median 70.876
Max 89.194
NumMissing 7
AverageOfOct_18: 13173×1 double
Properties:
Description: Average of Oct-18
Values:
Min 37.539
Median 57.454
Max 81.46
NumMissing 7
AverageOfNov_18: 13173×1 double
Properties:
Description: Average of Nov-18
Values:
Min 19.145
Median 42.426
Max 76.301
NumMissing 12
AverageOfDec_18: 13173×1 double
Properties:
Description: Average of Dec-18
Values:
Min 15.377
Median 38.496
Max 73.539
NumMissing 33
metastatic_diagnosis_period: 13173×1 double
Properties:
Description: metastatic_diagnosis_period
Values:
Min 0
Median 44
Max 365
- There are a lot of rows or variables that just say “cell array of character vectors”, which doesn’t tell us much about the data.
- There are a few variables that have a high ‘NumMissing’ value.
- The numeric variables can have dramatically different minimums and maximums.
Process and Clean the Data
1. Convert text data to categorical
2. Handle Missing Data
patient_id | payer_type | patient_state | patient_zip3 | Region | Division | patient_age | patient_gender | breast_cancer_diagnosis_code | breast_cancer_diagnosis_desc | metastatic_cancer_diagnosis_code | population | density | age_median | age_under_10 | age_10_to_19 | age_20s | age_30s | age_40s | age_50s | age_60s | age_70s | age_over_80 | male | female | married | divorced | never_married | widowed | family_size | |
---|---|---|---|---|---|---|---|---|---|---|---|---|---|---|---|---|---|---|---|---|---|---|---|---|---|---|---|---|---|---|
1 | 268700 | COMMERCIAL | AR | 724 | South | West South Central | 39 | F | C50912 | Malignant neoplasm of unspecified site of left female breast | C773 | 3.9249e+03 | 82.6283 | 42.5750 | 11.6050 | 13.0317 | 10.8667 | 11.8017 | 12.2917 | 13.2167 | 13.4717 | 10.0717 | 3.6350 | 51.4317 | 48.5683 | 51.0483 | 16.7233 | 23.5650 | 8.6550 | 3.0093 |
2 | 484983 | <undefined> | IL | 629 | Midwest | East North Central | 55 | F | C50412 | Malig neoplasm of upper-outer quadrant of left female breast | C773 | 2.7454e+03 | 51.7936 | 43.5351 | 11.2247 | 12.1922 | 11.4467 | 11.0065 | 11.3545 | 14.3922 | 14.1507 | 9.1727 | 5.0506 | 49.3234 | 50.6766 | 49.4753 | 15.4182 | 26.9286 | 8.1714 | 3.1749 |
3 | 277055 | COMMERCIAL | CA | undefined | West | Pacific | 59 | F | 1749 | Malignant neoplasm of breast (female), unspecified | C773 | 3.8343e+04 | 700.3375 | 36.2795 | 13.2667 | 15.6641 | 13.4949 | 13.4538 | 12.4000 | 11.5846 | 10.4667 | 6.3769 | 3.2846 | 49.9897 | 50.0103 | 48.8077 | 11.8974 | 34.3487 | 4.9487 | 3.7977 |
4 | 320055 | MEDICAID | CA | 925 | West | Pacific | 59 | F | C50911 | Malignant neoplasm of unsp site of right female breast | C773 | 3.6054e+04 | 5.2943e+03 | 36.6538 | 9.7615 | 11.2677 | 17.2339 | 17.4415 | 13.0908 | 12.3046 | 9.4077 | 5.6738 | 3.8246 | 50.5108 | 49.4892 | 33.4785 | 11.3015 | 50.4569 | 4.7662 | 3.4429 |
5 | 190386 | COMMERCIAL | CA | 934 | West | Pacific | 71 | F | 1748 | Malignant neoplasm of other specified sites of female breast | C7951 | 1.3700e+04 | 400.4763 | 41.7816 | 10.0316 | 16.4342 | 12.9710 | 11.2921 | 10.0868 | 11.5605 | 13.2790 | 8.7842 | 5.5316 | 51.9895 | 48.0132 | 48.2079 | 11.1632 | 35.6026 | 5.0132 | 3.0909 |
6 | 559027 | COMMERCIAL | IN | 461 | Midwest | East North Central | 63 | F | 1749 | Malignant neoplasm of breast (female), unspecified | C786 | 9.3229e+03 | 274.7371 | 40.1237 | 12.2300 | 13.8800 | 11.5317 | 11.9350 | 12.5517 | 13.9117 | 13.0467 | 7 | 3.9067 | 50.9817 | 49.0183 | 57.1617 | 12.7767 | 23.5267 | 6.5333 | 3.1912 |
7 | 293747 | MEDICARE ADVANTAGE | OH | 448 | Midwest | East North Central | 57 | F | C50412 | Malig neoplasm of upper-outer quadrant of left female breast | C799 | 5.8906e+03 | 122.3929 | 42.4536 | 12.4286 | 13.1893 | 10.8089 | 10.7321 | 13.0411 | 13.2786 | 14.2804 | 7.5732 | 4.6786 | 49.9107 | 50.0893 | 55.8696 | 12.4232 | 24.4518 | 7.2625 | 2.9912 |
8 | 517596 | COMMERCIAL | DE | 198 | South | South Atlantic | 56 | F | C50411 | Malig neoplm of upper-outer quadrant of right female breast | C792 | 2.2036e+04 | 1.4505e+03 | 41.6300 | 11.0300 | 11.9800 | 12.1100 | 13.6900 | 11.6600 | 13.9500 | 12.9700 | 7.6900 | 4.9700 | 47.8200 | 52.1800 | 42.0300 | 13.3700 | 38.5100 | 6.1000 | 3.0850 |
9 | 533188 | COMMERCIAL | LA | 706 | South | West South Central | 65 | F | C50212 | Malig neoplasm of upper-inner quadrant of left female breast | C773 | 7.2198e+03 | 531.0590 | 39.5421 | 12.4474 | 14.7868 | 11.0026 | 12.5368 | 11.6868 | 14.6947 | 12.4789 | 5.8816 | 4.4947 | 50.5500 | 49.4500 | 49.0737 | 17.2132 | 27.2553 | 6.4711 | 3.2531 |
10 | 639484 | COMMERCIAL | CA | 922 | West | Pacific | 60 | F | C50912 | Malignant neoplasm of unspecified site of left female breast | C773 | 1.6550e+04 | 245.0979 | 44.2326 | 9.8872 | 10.4149 | 13.6723 | 11.3894 | 9.1447 | 15.5638 | 14.6277 | 10.1106 | 5.2298 | 54.2000 | 45.8000 | 46.5192 | 13.1872 | 33.9894 | 6.2957 | 3.3669 |
11 | 366431 | MEDICARE ADVANTAGE | PA | 191 | Northeast | Middle Atlantic | 71 | F | C50911 | Malignant neoplasm of unsp site of right female breast | C7989 | 3.1948e+04 | 5.5122e+03 | 35.7191 | 10.8532 | 10.9511 | 18.1596 | 17.3489 | 11.6468 | 11.0979 | 10.6425 | 5.9426 | 3.3511 | 48.3085 | 51.6915 | 32.4915 | 12.3021 | 49.7702 | 5.4298 | 3.0866 |
12 | 793091 | MEDICARE ADVANTAGE | OH | 453 | Midwest | East North Central | 73 | F | C50811 | Malignant neoplasm of ovrlp sites of right female breast | C773 | 6.4682e+03 | 196.6312 | 40.5818 | 12.2182 | 14.1455 | 12.2714 | 11.5338 | 12.0546 | 13.7792 | 12.8234 | 7.2948 | 3.8766 | 50.0740 | 49.9260 | 56.5662 | 11.9610 | 25.8195 | 5.6506 | 3.0866 |
13 | 942172 | MEDICARE ADVANTAGE | MN | 553 | Midwest | West North Central | 73 | F | 1749 | Malignant neoplasm of breast (female), unspecified | C773 | 1.2190e+04 | 249.1628 | 40.7686 | 12.8465 | 14.0198 | 10.0698 | 12.7035 | 12.9919 | 14.9977 | 12.1826 | 6.5198 | 3.6779 | 50.9942 | 49.0058 | 58.1977 | 10.6512 | 26.5395 | 4.6081 | 3.1352 |
14 | 834862 | COMMERCIAL | MI | 481 | Midwest | East North Central | 47 | F | 1749 | Malignant neoplasm of breast (female), unspecified | C773 | 2.3266e+04 | 743.5571 | 41.4729 | 10.9443 | 13.5914 | 12.6671 | 11.6100 | 12.1371 | 14.6457 | 12.7271 | 7.9286 | 3.7514 | 49.4800 | 50.5200 | 50.2657 | 11.7486 | 32.4871 | 5.5043 | 3.1332 |
⋮ |
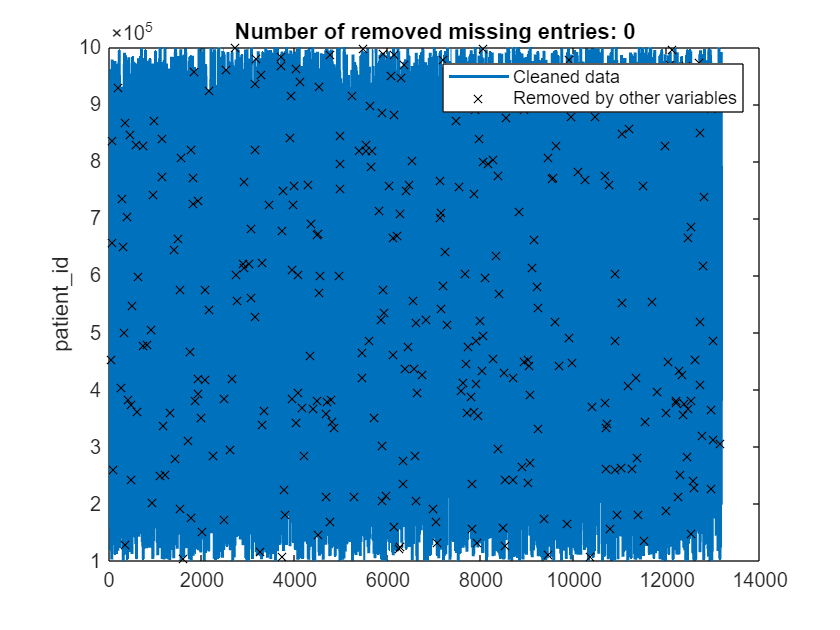
Explore the Data
Visual Analysis – Univariate Data

Visual Analysis – Bivariate Data

Statistical Analysis
patient_id | payer_type | patient_state | patient_zip3 | Region | Division | patient_age | patient_gender | breast_cancer_diagnosis_code | breast_cancer_diagnosis_desc | metastatic_cancer_diagnosis_code | population | density | age_median | age_under_10 | age_10_to_19 | age_20s | age_30s | age_40s | age_50s | age_60s | age_70s | age_over_80 | male | female | married | divorced | never_married | widowed | family_size | |
---|---|---|---|---|---|---|---|---|---|---|---|---|---|---|---|---|---|---|---|---|---|---|---|---|---|---|---|---|---|---|
1 | 268700 | COMMERCIAL | AR | 724 | South | West South Central | 39 | F | C50912 | Malignant neoplasm of unspecified site of left female breast | C773 | 3.9249e+03 | 82.6283 | 42.5750 | 11.6050 | 13.0317 | 10.8667 | 11.8017 | 12.2917 | 13.2167 | 13.4717 | 10.0717 | 3.6350 | 51.4317 | 48.5683 | 51.0483 | 16.7233 | 23.5650 | 8.6550 | 3.0093 |
2 | 484983 | <undefined> | IL | 629 | Midwest | East North Central | 55 | F | C50412 | Malig neoplasm of upper-outer quadrant of left female breast | C773 | 2.7454e+03 | 51.7936 | 43.5351 | 11.2247 | 12.1922 | 11.4467 | 11.0065 | 11.3545 | 14.3922 | 14.1507 | 9.1727 | 5.0506 | 49.3234 | 50.6766 | 49.4753 | 15.4182 | 26.9286 | 8.1714 | 3.1749 |
3 | 277055 | COMMERCIAL | CA | 925 | West | Pacific | 59 | F | 1749 | Malignant neoplasm of breast (female), unspecified | C773 | 3.8343e+04 | 700.3375 | 36.2795 | 13.2667 | 15.6641 | 13.4949 | 13.4538 | 12.4000 | 11.5846 | 10.4667 | 6.3769 | 3.2846 | 49.9897 | 50.0103 | 48.8077 | 11.8974 | 34.3487 | 4.9487 | 3.7977 |
4 | 320055 | MEDICAID | CA | 900 | West | Pacific | 59 | F | C50911 | Malignant neoplasm of unsp site of right female breast | C773 | 3.6054e+04 | 5.2943e+03 | 36.6538 | 9.7615 | 11.2677 | 17.2339 | 17.4415 | 13.0908 | 12.3046 | 9.4077 | 5.6738 | 3.8246 | 50.5108 | 49.4892 | 33.4785 | 11.3015 | 50.4569 | 4.7662 | 3.4429 |
5 | 190386 | COMMERCIAL | CA | 934 | West | Pacific | 71 | F | 1748 | Malignant neoplasm of other specified sites of female breast | C7951 | 1.3700e+04 | 400.4763 | 41.7816 | 10.0316 | 16.4342 | 12.9710 | 11.2921 | 10.0868 | 11.5605 | 13.2790 | 8.7842 | 5.5316 | 51.9895 | 48.0132 | 48.2079 | 11.1632 | 35.6026 | 5.0132 | 3.0909 |
6 | 559027 | COMMERCIAL | IN | 461 | Midwest | East North Central | 63 | F | 1749 | Malignant neoplasm of breast (female), unspecified | C786 | 9.3229e+03 | 274.7371 | 40.1237 | 12.2300 | 13.8800 | 11.5317 | 11.9350 | 12.5517 | 13.9117 | 13.0467 | 7 | 3.9067 | 50.9817 | 49.0183 | 57.1617 | 12.7767 | 23.5267 | 6.5333 | 3.1912 |
7 | 293747 | MEDICARE ADVANTAGE | OH | 448 | Midwest | East North Central | 57 | F | C50412 | Malig neoplasm of upper-outer quadrant of left female breast | C799 | 5.8906e+03 | 122.3929 | 42.4536 | 12.4286 | 13.1893 | 10.8089 | 10.7321 | 13.0411 | 13.2786 | 14.2804 | 7.5732 | 4.6786 | 49.9107 | 50.0893 | 55.8696 | 12.4232 | 24.4518 | 7.2625 | 2.9912 |
8 | 517596 | COMMERCIAL | DE | 198 | South | South Atlantic | 56 | F | C50411 | Malig neoplm of upper-outer quadrant of right female breast | C792 | 2.2036e+04 | 1.4505e+03 | 41.6300 | 11.0300 | 11.9800 | 12.1100 | 13.6900 | 11.6600 | 13.9500 | 12.9700 | 7.6900 | 4.9700 | 47.8200 | 52.1800 | 42.0300 | 13.3700 | 38.5100 | 6.1000 | 3.0850 |
9 | 533188 | COMMERCIAL | LA | 706 | South | West South Central | 65 | F | C50212 | Malig neoplasm of upper-inner quadrant of left female breast | C773 | 7.2198e+03 | 531.0590 | 39.5421 | 12.4474 | 14.7868 | 11.0026 | 12.5368 | 11.6868 | 14.6947 | 12.4789 | 5.8816 | 4.4947 | 50.5500 | 49.4500 | 49.0737 | 17.2132 | 27.2553 | 6.4711 | 3.2531 |
10 | 639484 | COMMERCIAL | CA | 922 | West | Pacific | 60 | F | C50912 | Malignant neoplasm of unspecified site of left female breast | C773 | 1.6550e+04 | 245.0979 | 44.2326 | 9.8872 | 10.4149 | 13.6723 | 11.3894 | 9.1447 | 15.5638 | 14.6277 | 10.1106 | 5.2298 | 54.2000 | 45.8000 | 46.5192 | 13.1872 | 33.9894 | 6.2957 | 3.3669 |
11 | 366431 | MEDICARE ADVANTAGE | PA | 191 | Northeast | Middle Atlantic | 71 | F | C50911 | Malignant neoplasm of unsp site of right female breast | C7989 | 3.1948e+04 | 5.5122e+03 | 35.7191 | 10.8532 | 10.9511 | 18.1596 | 17.3489 | 11.6468 | 11.0979 | 10.6425 | 5.9426 | 3.3511 | 48.3085 | 51.6915 | 32.4915 | 12.3021 | 49.7702 | 5.4298 | 3.0866 |
12 | 793091 | MEDICARE ADVANTAGE | OH | 453 | Midwest | East North Central | 73 | F | C50811 | Malignant neoplasm of ovrlp sites of right female breast | C773 | 6.4682e+03 | 196.6312 | 40.5818 | 12.2182 | 14.1455 | 12.2714 | 11.5338 | 12.0546 | 13.7792 | 12.8234 | 7.2948 | 3.8766 | 50.0740 | 49.9260 | 56.5662 | 11.9610 | 25.8195 | 5.6506 | 3.0866 |
13 | 942172 | MEDICARE ADVANTAGE | MN | 553 | Midwest | West North Central | 73 | F | 1749 | Malignant neoplasm of breast (female), unspecified | C773 | 1.2190e+04 | 249.1628 | 40.7686 | 12.8465 | 14.0198 | 10.0698 | 12.7035 | 12.9919 | 14.9977 | 12.1826 | 6.5198 | 3.6779 | 50.9942 | 49.0058 | 58.1977 | 10.6512 | 26.5395 | 4.6081 | 3.1352 |
14 | 834862 | COMMERCIAL | MI | 481 | Midwest | East North Central | 47 | F | 1749 | Malignant neoplasm of breast (female), unspecified | C773 | 2.3266e+04 | 743.5571 | 41.4729 | 10.9443 | 13.5914 | 12.6671 | 11.6100 | 12.1371 | 14.6457 | 12.7271 | 7.9286 | 3.7514 | 49.4800 | 50.5200 | 50.2657 | 11.7486 | 32.4871 | 5.5043 | 3.1332 |
⋮ |
Feature Engineering
FeatureTransformer with properties:
Type: ‘regression’
TargetLearner: ‘linear’
NumEngineeredFeatures: 28
NumOriginalFeatures: 2
TotalNumFeatures: 30
breast_cancer_diagnosis_code | breast_cancer_diagnosis_desc | zsc(cos(yearsFromMeanAge)) | zsc(health_uninsured.*yearsFromMeanAge) | zsc(AverageOfJan_14-AverageOfFeb_14) | zsc(AverageOfOct_16./AverageOfApr_17) | zsc(AverageOfJan_13./AverageOfDec_16) | eb11(patient_age) | eb11(yearsFromMeanAge) | zsc(sin(AverageOfNov_18)) | zsc(labor_force_participation+disabled) | zsc(cos(AverageOfJun_13)) | zsc(sin(AverageOfOct_18)) | zsc(patient_age./hispanic) | zsc(sin(age_20s)) | zsc(cos(AverageOfJul_15)) | zsc(yearsFromMeanAge.^2) | zsc(farmer.*yearsFromMeanAge) | zsc(sig(patient_age)) | eb24(income_household_100_to_150) | zsc(cos(AverageOfDec_17)) | zsc(cos(rent_median)) | zsc(tanh(age_40s)) | zsc(race_black.*race_pacific) | eb28(education_graduate) | zsc(cos(AverageOfAug_18)) | zsc(AverageOfMar_13./AverageOfFeb_16) | zsc(sin(AverageOfNov_13)) | zsc(cos(AverageOfNov_18)) | zsc(health_uninsured./yearsFromMeanAge) | |
---|---|---|---|---|---|---|---|---|---|---|---|---|---|---|---|---|---|---|---|---|---|---|---|---|---|---|---|---|---|---|
1 | C50912 | Malignant neoplasm of unspecified site of left female breast | 0.1806 | -1.2893 | 0.0690 | -0.3920 | 0.0890 | 2 | 2 | -1.2710 | 0.1981 | 1.0835 | -0.4009 | 0.2163 | -1.1510 | 1.2808 | 0.9764 | -2.2388 | 0.0280 | 1 | 0.6620 | 1.1890 | 0.0776 | -0.4848 | 1 | -1.2617 | -0.5500 | 1.3750 | 0.9595 | 0.0631 |
2 | C50412 | Malig neoplasm of upper-outer quadrant of left female breast | -0.6655 | -0.2150 | 0.2483 | -0.0630 | 0.3927 | 5 | 5 | 1.2637 | -0.6535 | 0.0614 | 1.3875 | 0.6865 | -1.0175 | -1.3784 | -0.6453 | -0.4617 | 0.0280 | 4 | -1.0882 | -1.0191 | 0.0684 | -0.3514 | 3 | 1.5636 | -0.2443 | -1.2160 | -0.3726 | -0.0954 |
3 | 1749 | Malignant neoplasm of breast (female), unspecified | 1.3154 | 0.0054 | 0.5232 | 0.1254 | -0.1329 | 6 | 6 | -0.2890 | -0.7538 | -0.7796 | 1.4197 | -0.5775 | 1.4565 | 1.0324 | -0.7200 | -0.0024 | 0.0280 | 20 | -1.2077 | -0.6610 | 0.0780 | 0.6752 | 6 | -0.9565 | -0.2047 | -0.9773 | 1.5936 | -3.9151 |
4 | C50911 | Malignant neoplasm of unsp site of right female breast | 1.3154 | 6.9626e-04 | 1.5665 | 0.4103 | 0.3231 | 6 | 6 | 0.6535 | 0.3172 | 0.9866 | -0.2370 | -0.5749 | -1.1616 | -1.3856 | -0.7200 | -8.3799e-05 | 0.0280 | 9 | -1.0435 | 0.2634 | 0.0790 | 0.3610 | 13 | 1.6817 | -1.8093 | 1.1615 | -1.0371 | -5.0481 |
5 | 1748 | Malignant neoplasm of other specified sites of female breast | 0.9097 | 0.6396 | 1.2400 | 0.3110 | 0.0649 | 9 | 9 | -1.2927 | -2.1295 | -0.1996 | 0.8063 | -0.5212 | 0.8644 | 0.3204 | -0.1503 | 0.3570 | 0.0280 | 17 | -0.9154 | -0.3898 | -0.0585 | -0.0649 | 11 | 0.9956 | -1.1728 | 1.4639 | -0.5341 | 0.1862 |
6 | 1749 | Malignant neoplasm of breast (female), unspecified | -1.2099 | 0.1862 | 5.6886e-04 | -0.5225 | 0.1049 | 7 | 7 | -0.8391 | 0.7661 | -0.8233 | -1.2811 | 1.4600 | -0.9590 | -0.9225 | -0.6624 | 0.3039 | 0.0280 | 21 | -0.3768 | -1.0583 | 0.0783 | -0.5007 | 4 | 1.2107 | 0.1415 | 1.4069 | 1.3855 | 0.3022 |
7 | C50412 | Malig neoplasm of upper-outer quadrant of left female breast | -0.9412 | -0.1142 | 0.0602 | -0.3997 | 0.1448 | 6 | 6 | -1.4337 | 0.4192 | 1.2975 | -0.1379 | 0.4657 | -1.1378 | -0.3066 | -0.6992 | -0.2427 | 0.0280 | 13 | -0.8261 | -1.3789 | 0.0790 | -0.4774 | 1 | 0.3635 | 0.2951 | 0.3963 | 0.6159 | -0.3145 |
8 | C50411 | Malig neoplm of upper-outer quadrant of right female breast | -1.4468 | -0.0944 | -0.3012 | -1.2779 | -0.1211 | 6 | 6 | -0.1400 | 0.6606 | -1.2905 | 0.1472 | -0.1780 | -0.3494 | 0.1091 | -0.6764 | -4.9683e-05 | 0.0280 | 14 | -1.1083 | -1.2292 | 0.0734 | -0.3837 | 20 | 1.2717 | 1.6932 | 0.2868 | -1.2188 | -0.0616 |
9 | C50212 | Malig neoplasm of upper-inner quadrant of left female breast | 1.1607 | 0.3906 | -1.0540 | -0.6968 | -0.2237 | 7 | 8 | -0.4944 | -0.7009 | 1.2929 | 0.9208 | 1.0272 | -1.1630 | -0.6645 | -0.5839 | 0.5773 | 0.0280 | 9 | 0.8457 | 1.2404 | 0.0737 | 1.5897 | 1 | 1.2651 | -0.1321 | -0.8378 | 1.5453 | 0.2936 |
10 | C50912 | Malignant neoplasm of unspecified site of left female breast | 0.9918 | 0.0709 | -0.2100 | 0.4588 | -0.4328 | 6 | 6 | -1.1849 | -1.6220 | -1.0465 | 1.4286 | -0.5794 | 1.5920 | 0.4326 | -0.7180 | 0.0020 | 0.0280 | 4 | 1.3263 | 0.4417 | -0.8275 | -0.1101 | 4 | -0.1867 | 0.4845 | -1.2424 | -0.6868 | 1.5358 |
11 | C50911 | Malignant neoplasm of unsp site of right female breast | 0.9097 | 0.6159 | -0.2138 | -1.4477 | -0.7481 | 9 | 9 | -0.8974 | 1.3344 | -1.2279 | -0.0286 | -0.2587 | -0.6343 | 0.9810 | -0.1503 | -4.9683e-05 | 0.0280 | 7 | -1.2504 | 1.3439 | 0.0732 | 0.1566 | 17 | -0.6010 | 1.1877 | -0.2407 | -0.9555 | 0.1834 |
12 | C50811 | Malignant neoplasm of ovrlp sites of right female breast | 0.4955 | 0.5783 | -0.3673 | -0.4973 | 0.3141 | 9 | 9 | -1.2544 | 0.7968 | 1.1712 | -1.4259 | 1.4166 | -0.1312 | -0.4201 | 0.0604 | 1.0940 | 0.0280 | 17 | -1.2310 | -0.8610 | 0.0766 | -0.4922 | 5 | 0.5016 | 0.1765 | 1.2579 | 0.9843 | 0.1616 |
13 | 1749 | Malignant neoplasm of breast (female), unspecified | 0.4955 | 0.4398 | 0.5914 | 0.1312 | -1.7375 | 9 | 9 | 0.8544 | 1.4799 | -1.2113 | -0.9255 | 0.5154 | -0.5829 | -0.2644 | 0.0604 | 1.1872 | 0.0280 | 24 | -0.3583 | 0.8771 | 0.0789 | -0.5004 | 8 | 1.0030 | 0.6482 | -1.0818 | 1.2931 | 0.1498 |
14 | 1749 | Malignant neoplasm of breast (female), unspecified | 1.2957 | -0.4079 | -0.3742 | -0.0806 | -0.1207 | 4 | 4 | 0.5078 | -0.2391 | 0.3034 | -0.6520 | 0.0119 | 0.4380 | 0.9356 | -0.0990 | -0.1350 | 0.0280 | 16 | 1.3104 | -1.4723 | 0.0770 | -0.4757 | 13 | -1.2384 | 0.6673 | -1.2145 | -1.1079 | 0.0685 |
⋮ |
___________ __________ ___________________________________ ______________________________________________________________breast_cancer_diagnosis_code Categorical true breast_cancer_diagnosis_code
breast_cancer_diagnosis_desc Categorical true breast_cancer_diagnosis_desc
zsc(cos(yearsFromMeanAge)) Numeric false yearsFromMeanAge cos( )
Standardization with z-score (mean = 0.03342, std = 0.70961)
zsc(health_uninsured.*yearsFromMeanAge) Numeric false health_uninsured, yearsFromMeanAge health_uninsured .* yearsFromMeanAge
Standardization with z-score (mean = -2.7558, std = 124.453)
zsc(AverageOfJan_14-AverageOfFeb_14) Numeric false AverageOfJan_14, AverageOfFeb_14 AverageOfJan_14 – AverageOfFeb_14
Standardization with z-score (mean = -2.4227, std = 3.8007)
zsc(AverageOfOct_16./AverageOfApr_17) Numeric false AverageOfOct_16, AverageOfApr_17 AverageOfOct_16 ./ AverageOfApr_17
Standardization with z-score (mean = 1.0531, std = 0.040559)
zsc(AverageOfJan_13./AverageOfDec_16) Numeric false AverageOfJan_13, AverageOfDec_16 AverageOfJan_13 ./ AverageOfDec_16
Standardization with z-score (mean = 0.96755, std = 0.07866)
eb11(patient_age) Categorical false patient_age Equal-width binning (number of bins = 11)
eb11(yearsFromMeanAge) Categorical false yearsFromMeanAge Equal-width binning (number of bins = 11)
zsc(sin(AverageOfNov_18)) Numeric false AverageOfNov_18 sin( )
Standardization with z-score (mean = 0.039513, std = 0.69365)
zsc(labor_force_participation+disabled) Numeric false labor_force_participation, disabled labor_force_participation + disabled
Standardization with z-score (mean = 75.1061, std = 3.7296)
zsc(cos(AverageOfJun_13)) Numeric false AverageOfJun_13 cos( )
Standardization with z-score (mean = 0.014056, std = 0.75911)
zsc(sin(AverageOfOct_18)) Numeric false AverageOfOct_18 sin( )
Standardization with z-score (mean = -0.00117, std = 0.70011)
zsc(patient_age./hispanic) Numeric false patient_age, hispanic patient_age ./ hispanic
Standardization with z-score (mean = 9.7121, std = 14.6393)
zsc(sin(age_20s)) Numeric false age_20s sin( )
Standardization with z-score (mean = -0.20048, std = 0.68741)
zsc(cos(AverageOfJul_15)) Numeric false AverageOfJul_15 cos( )
Standardization with z-score (mean = 0.012229, std = 0.72983)
zsc(yearsFromMeanAge.^2) Numeric false yearsFromMeanAge power( ,2)
Standardization with z-score (mean = 174.2181, std = 241.8873)
zsc(farmer.*yearsFromMeanAge) Numeric false farmer, yearsFromMeanAge farmer .* yearsFromMeanAge
Standardization with z-score (mean = 0.0023864, std = 48.0329)
zsc(sig(patient_age)) Numeric false patient_age sigmoid( )
Standardization with z-score (mean = 1, std = 2.6634e-10)
eb24(income_household_100_to_150) Categorical false income_household_100_to_150 Equal-width binning (number of bins = 24)
zsc(cos(AverageOfDec_17)) Numeric false AverageOfDec_17 cos( )
Standardization with z-score (mean = -0.0045992, std = 0.7565)
zsc(cos(rent_median)) Numeric false rent_median cos( )
Standardization with z-score (mean = 0.053355, std = 0.69262)
zsc(tanh(age_40s)) Numeric false age_40s tanh( )
Standardization with z-score (mean = 1, std = 2.5149e-08)
zsc(race_black.*race_pacific) Numeric false race_black, race_pacific race_black .* race_pacific
Standardization with z-score (mean = 1.0419, std = 2.0598)
eb28(education_graduate) Categorical false education_graduate Equal-width binning (number of bins = 28)
zsc(cos(AverageOfAug_18)) Numeric false AverageOfAug_18 cos( )
Standardization with z-score (mean = -0.13184, std = 0.66549)
zsc(AverageOfMar_13./AverageOfFeb_16) Numeric false AverageOfMar_13, AverageOfFeb_16 AverageOfMar_13 ./ AverageOfFeb_16
Standardization with z-score (mean = 1.0327, std = 0.065144)
zsc(sin(AverageOfNov_13)) Numeric false AverageOfNov_13 sin( )
Standardization with z-score (mean = -0.075478, std = 0.73244)
zsc(cos(AverageOfNov_18)) Numeric false AverageOfNov_18 cos( )
Standardization with z-score (mean = -0.13799, std = 0.70592)
zsc(health_uninsured./yearsFromMeanAge) Numeric false health_uninsured, yearsFromMeanAge health_uninsured ./ yearsFromMeanAge
Standardization with z-score (mean = -0.88776, std = 7.7614)
Train a Machine Learning Model
Total iterations (MaxObjectiveEvaluations): 255
Total time (MaxTime): Inf|=============================================================================================================================================|
| Iter | Eval | log(1+valLoss)| Time for training | Observed min | Training set | Learner | Hyperparameter: Value |
| | result | | & validation (sec)| validation loss | size | | |
|=============================================================================================================================================|
| 1 | Best | 9.3862 | 1.0312 | 9.3862 | 161 | tree | MinLeafSize: 173 |
| 2 | Best | 9.384 | 2.092 | 9.384 | 161 | ensemble | Method: LSBoost |
| | | | | | | | NumLearningCycles: 232 |
| | | | | | | | MinLeafSize: 1084 |
| 3 | Accept | 38.104 | 0.45806 | 9.384 | 161 | svm | BoxConstraint: 0.011812 |
| | | | | | | | KernelScale: 2.2883 |
| | | | | | | | Epsilon: 38.982 |
| 4 | Best | 9.3825 | 0.28987 | 9.3825 | 161 | tree | MinLeafSize: 140 |
| 5 | Best | 8.9046 | 0.21544 | 8.9046 | 643 | tree | MinLeafSize: 140 |
| 6 | Accept | 9.3853 | 0.10889 | 8.9046 | 161 | tree | MinLeafSize: 5183 |
| 7 | Accept | 8.9281 | 0.10868 | 8.9046 | 161 | tree | MinLeafSize: 45 |
| 8 | Accept | 63.931 | 0.48431 | 8.9046 | 161 | svm | BoxConstraint: 0.0032309 |
| | | | | | | | KernelScale: 4.7109 |
| | | | | | | | Epsilon: 8.986 |
| 9 | Accept | 45.964 | 4.3819 | 8.9046 | 161 | svm | BoxConstraint: 0.12087 |
| | | | | | | | KernelScale: 0.088521 |
| | | | | | | | Epsilon: 0.97865 |
| 10 | Accept | 8.94 | 0.067605 | 8.9046 | 643 | tree | MinLeafSize: 45 |
| 11 | Accept | 71.49 | 4.8778 | 8.9046 | 161 | svm | BoxConstraint: 317.32 |
| | | | | | | | KernelScale: 0.010993 |
| | | | | | | | Epsilon: 19.065 |
| 12 | Accept | 9.3893 | 0.16924 | 8.9046 | 161 | svm | BoxConstraint: 0.11231 |
| | | | | | | | KernelScale: 34.956 |
| | | | | | | | Epsilon: 4417.5 |
| 13 | Accept | 9.3273 | 0.051708 | 8.9046 | 161 | tree | MinLeafSize: 33 |
| 14 | Accept | 9.4163 | 0.061619 | 8.9046 | 161 | svm | BoxConstraint: 0.12262 |
| | | | | | | | KernelScale: 16.877 |
| | | | | | | | Epsilon: 539.11 |
| 15 | Accept | 8.9338 | 0.066245 | 8.9046 | 643 | tree | MinLeafSize: 33 |
| 16 | Accept | 43.881 | 0.082721 | 8.9046 | 161 | svm | BoxConstraint: 49.319 |
| | | | | | | | KernelScale: 4.6223 |
| | | | | | | | Epsilon: 59.775 |
| 17 | Accept | 9.4191 | 0.057352 | 8.9046 | 161 | svm | BoxConstraint: 0.16688 |
| | | | | | | | KernelScale: 0.0023583 |
| | | | | | | | Epsilon: 1744.5 |
| 18 | Accept | 9.393 | 0.058098 | 8.9046 | 161 | svm | BoxConstraint: 0.17661 |
| | | | | | | | KernelScale: 0.0014019 |
| | | | | | | | Epsilon: 872.82 |
| 19 | Accept | 9.2595 | 0.058433 | 8.9046 | 161 | tree | MinLeafSize: 3 |
| 20 | Accept | 9.3043 | 0.085948 | 8.9046 | 643 | tree | MinLeafSize: 3 |
|=============================================================================================================================================|
| Iter | Eval | log(1+valLoss)| Time for training | Observed min | Training set | Learner | Hyperparameter: Value |
| | result | | & validation (sec)| validation loss | size | | |
|=============================================================================================================================================|
| 21 | Best | 8.8832 | 0.080987 | 8.8832 | 2569 | tree | MinLeafSize: 140 |
| 22 | Accept | 9.4095 | 0.061143 | 8.8832 | 161 | svm | BoxConstraint: 0.018037 |
| | | | | | | | KernelScale: 61.209 |
| | | | | | | | Epsilon: 256.27 |
| 23 | Accept | 9.4198 | 0.057961 | 8.8832 | 161 | svm | BoxConstraint: 0.11446 |
| | | | | | | | KernelScale: 15.272 |
| | | | | | | | Epsilon: 197.66 |
| 24 | Accept | 9.1218 | 0.050487 | 8.8832 | 161 | tree | MinLeafSize: 34 |
| 25 | Accept | 9.3875 | 1.7749 | 8.8832 | 161 | ensemble | Method: Bag |
| | | | | | | | NumLearningCycles: 294 |
| | | | | | | | MinLeafSize: 273 |
| 26 | Accept | 8.9284 | 0.11047 | 8.8832 | 643 | tree | MinLeafSize: 34 |
| 27 | Accept | 9.3863 | 0.052435 | 8.8832 | 161 | tree | MinLeafSize: 2719 |
| 28 | Accept | 9.4099 | 0.065684 | 8.8832 | 161 | svm | BoxConstraint: 0.011394 |
| | | | | | | | KernelScale: 0.0018703 |
| | | | | | | | Epsilon: 3.3641 |
| 29 | Accept | 12.819 | 0.5708 | 8.8832 | 161 | svm | BoxConstraint: 28.941 |
| | | | | | | | KernelScale: 6.0836 |
| | | | | | | | Epsilon: 31.22 |
| 30 | Accept | 67.346 | 0.58923 | 8.8832 | 161 | svm | BoxConstraint: 244.94 |
| | | | | | | | KernelScale: 8.5597 |
| | | | | | | | Epsilon: 2.3973 |
| 31 | Accept | 9.3879 | 1.4179 | 8.8832 | 643 | ensemble | Method: LSBoost |
| | | | | | | | NumLearningCycles: 232 |
| | | | | | | | MinLeafSize: 1084 |
| 32 | Accept | 65.415 | 0.091055 | 8.8832 | 161 | svm | BoxConstraint: 1.2417 |
| | | | | | | | KernelScale: 0.0050643 |
| | | | | | | | Epsilon: 76.681 |
| 33 | Accept | 9.4225 | 0.060363 | 8.8832 | 161 | svm | BoxConstraint: 0.0084308 |
| | | | | | | | KernelScale: 833.3 |
| | | | | | | | Epsilon: 730.67 |
| 34 | Accept | 8.9623 | 2.1224 | 8.8832 | 161 | ensemble | Method: Bag |
| | | | | | | | NumLearningCycles: 285 |
| | | | | | | | MinLeafSize: 12 |
| 35 | Accept | 50.056 | 0.56976 | 8.8832 | 161 | svm | BoxConstraint: 0.72025 |
| | | | | | | | KernelScale: 9.8778 |
| | | | | | | | Epsilon: 6.4438 |
| 36 | Best | 8.8771 | 2.5786 | 8.8771 | 643 | ensemble | Method: Bag |
| | | | | | | | NumLearningCycles: 285 |
| | | | | | | | MinLeafSize: 12 |
| 37 | Accept | 9.0976 | 0.084623 | 8.8771 | 161 | tree | MinLeafSize: 8 |
| 38 | Accept | 9.3819 | 1.4843 | 8.8771 | 161 | ensemble | Method: Bag |
| | | | | | | | NumLearningCycles: 294 |
| | | | | | | | MinLeafSize: 1255 |
| 39 | Accept | 8.9822 | 1.5021 | 8.8771 | 161 | ensemble | Method: Bag |
| | | | | | | | NumLearningCycles: 208 |
| | | | | | | | MinLeafSize: 16 |
| 40 | Accept | 45.349 | 0.50465 | 8.8771 | 161 | svm | BoxConstraint: 0.0031861 |
| | | | | | | | KernelScale: 1.1929 |
| | | | | | | | Epsilon: 3.846 |
|=============================================================================================================================================|
| Iter | Eval | log(1+valLoss)| Time for training | Observed min | Training set | Learner | Hyperparameter: Value |
| | result | | & validation (sec)| validation loss | size | | |
|=============================================================================================================================================|
| 41 | Best | 8.8726 | 1.7837 | 8.8726 | 643 | ensemble | Method: Bag |
| | | | | | | | NumLearningCycles: 208 |
| | | | | | | | MinLeafSize: 16 |
| 42 | Best | 8.8377 | 2.8179 | 8.8377 | 2569 | ensemble | Method: Bag |
| | | | | | | | NumLearningCycles: 208 |
| | | | | | | | MinLeafSize: 16 |
| 43 | Accept | 9.3834 | 1.4848 | 8.8377 | 161 | ensemble | Method: LSBoost |
| | | | | | | | NumLearningCycles: 269 |
| | | | | | | | MinLeafSize: 101 |
| 44 | Accept | 9.4026 | 0.075617 | 8.8377 | 161 | svm | BoxConstraint: 120.1 |
| | | | | | | | KernelScale: 1.5209 |
| | | | | | | | Epsilon: 1610.5 |
| 45 | Accept | 8.9233 | 1.5883 | 8.8377 | 161 | ensemble | Method: Bag |
| | | | | | | | NumLearningCycles: 224 |
| | | | | | | | MinLeafSize: 4 |
| 46 | Accept | 34.178 | 4.802 | 8.8377 | 161 | svm | BoxConstraint: 663.55 |
| | | | | | | | KernelScale: 0.045175 |
| | | | | | | | Epsilon: 3.6348 |
| 47 | Accept | 8.8728 | 1.9803 | 8.8377 | 643 | ensemble | Method: Bag |
| | | | | | | | NumLearningCycles: 224 |
| | | | | | | | MinLeafSize: 4 |
| 48 | Accept | 16.005 | 2.1964 | 8.8377 | 161 | svm | BoxConstraint: 41.536 |
| | | | | | | | KernelScale: 0.13288 |
| | | | | | | | Epsilon: 0.76209 |
| 49 | Accept | 9.5967 | 5.0388 | 8.8377 | 161 | svm | BoxConstraint: 434.82 |
| | | | | | | | KernelScale: 0.31522 |
| | | | | | | | Epsilon: 5.0709 |
| 50 | Accept | 9.4046 | 0.056141 | 8.8377 | 161 | svm | BoxConstraint: 0.0019764 |
| | | | | | | | KernelScale: 0.98483 |
| | | | | | | | Epsilon: 304.63 |
| 51 | Accept | 35.523 | 4.2078 | 8.8377 | 161 | svm | BoxConstraint: 0.017662 |
| | | | | | | | KernelScale: 0.0065272 |
| | | | | | | | Epsilon: 1.7329 |
| 52 | Accept | 9.1215 | 0.11814 | 8.8377 | 643 | tree | MinLeafSize: 8 |
| 53 | Accept | 9.3878 | 1.4928 | 8.8377 | 161 | ensemble | Method: LSBoost |
| | | | | | | | NumLearningCycles: 264 |
| | | | | | | | MinLeafSize: 2956 |
| 54 | Accept | 9.3846 | 0.054412 | 8.8377 | 161 | tree | MinLeafSize: 125 |
| 55 | Accept | 9.0298 | 0.053416 | 8.8377 | 161 | tree | MinLeafSize: 34 |
| 56 | Accept | 9.4066 | 0.067662 | 8.8377 | 161 | svm | BoxConstraint: 0.21675 |
| | | | | | | | KernelScale: 79.17 |
| | | | | | | | Epsilon: 764.21 |
| 57 | Accept | 8.942 | 0.07282 | 8.8377 | 643 | tree | MinLeafSize: 34 |
| 58 | Accept | 9.3883 | 1.3873 | 8.8377 | 161 | ensemble | Method: Bag |
| | | | | | | | NumLearningCycles: 267 |
| | | | | | | | MinLeafSize: 3998 |
| 59 | Accept | 9.1155 | 1.9223 | 8.8377 | 161 | ensemble | Method: LSBoost |
| | | | | | | | NumLearningCycles: 255 |
| | | | | | | | MinLeafSize: 7 |
| 60 | Accept | 11.03 | 0.093069 | 8.8377 | 161 | svm | BoxConstraint: 0.34881 |
| | | | | | | | KernelScale: 1.0691 |
| | | | | | | | Epsilon: 61.589 |
|=============================================================================================================================================|
| Iter | Eval | log(1+valLoss)| Time for training | Observed min | Training set | Learner | Hyperparameter: Value |
| | result | | & validation (sec)| validation loss | size | | |
|=============================================================================================================================================|
| 61 | Accept | 20.181 | 0.61277 | 8.8377 | 161 | svm | BoxConstraint: 82.516 |
| | | | | | | | KernelScale: 9.0767 |
| | | | | | | | Epsilon: 1.705 |
| 62 | Accept | 8.98 | 3.2622 | 8.8377 | 643 | ensemble | Method: LSBoost |
| | | | | | | | NumLearningCycles: 255 |
| | | | | | | | MinLeafSize: 7 |
| 63 | Accept | 8.8565 | 3.0996 | 8.8377 | 2569 | ensemble | Method: Bag |
| | | | | | | | NumLearningCycles: 224 |
| | | | | | | | MinLeafSize: 4 |
| 64 | Accept | 9.3866 | 1.0202 | 8.8377 | 161 | ensemble | Method: Bag |
| | | | | | | | NumLearningCycles: 207 |
| | | | | | | | MinLeafSize: 6286 |
| 65 | Accept | 31.849 | 0.56723 | 8.8377 | 161 | svm | BoxConstraint: 164.7 |
| | | | | | | | KernelScale: 977.44 |
| | | | | | | | Epsilon: 4.0873 |
| 66 | Accept | 9.3456 | 0.08663 | 8.8377 | 161 | tree | MinLeafSize: 3 |
| 67 | Accept | 9.3898 | 0.046713 | 8.8377 | 161 | tree | MinLeafSize: 568 |
| 68 | Accept | 9.3241 | 0.089116 | 8.8377 | 643 | tree | MinLeafSize: 3 |
| 69 | Accept | 25.765 | 0.62032 | 8.8377 | 161 | svm | BoxConstraint: 0.12804 |
| | | | | | | | KernelScale: 2.8982 |
| | | | | | | | Epsilon: 0.26435 |
| 70 | Accept | 9.392 | 0.061827 | 8.8377 | 161 | svm | BoxConstraint: 0.0036781 |
| | | | | | | | KernelScale: 135.24 |
| | | | | | | | Epsilon: 10235 |
| 71 | Accept | 65.542 | 4.5555 | 8.8377 | 161 | svm | BoxConstraint: 429.64 |
| | | | | | | | KernelScale: 0.1032 |
| | | | | | | | Epsilon: 140.83 |
| 72 | Accept | 9.6253 | 2.2298 | 8.8377 | 161 | svm | BoxConstraint: 28.772 |
| | | | | | | | KernelScale: 0.1677 |
| | | | | | | | Epsilon: 0.1355 |
| 73 | Accept | 9.3874 | 1.5589 | 8.8377 | 643 | ensemble | Method: Bag |
| | | | | | | | NumLearningCycles: 294 |
| | | | | | | | MinLeafSize: 1255 |
| 74 | Accept | 51.726 | 4.4621 | 8.8377 | 161 | svm | BoxConstraint: 210.52 |
| | | | | | | | KernelScale: 0.03399 |
| | | | | | | | Epsilon: 143.6 |
| 75 | Accept | 8.9997 | 2.0089 | 8.8377 | 161 | ensemble | Method: Bag |
| | | | | | | | NumLearningCycles: 281 |
| | | | | | | | MinLeafSize: 4 |
| 76 | Accept | 9.4831 | 4.4187 | 8.8377 | 161 | svm | BoxConstraint: 12.41 |
| | | | | | | | KernelScale: 0.046831 |
| | | | | | | | Epsilon: 0.18991 |
| 77 | Accept | 9.2437 | 0.063799 | 8.8377 | 161 | tree | MinLeafSize: 65 |
| 78 | Accept | 8.8737 | 2.5522 | 8.8377 | 643 | ensemble | Method: Bag |
| | | | | | | | NumLearningCycles: 281 |
| | | | | | | | MinLeafSize: 4 |
| 79 | Accept | 9.3952 | 1.5512 | 8.8377 | 161 | ensemble | Method: LSBoost |
| | | | | | | | NumLearningCycles: 274 |
| | | | | | | | MinLeafSize: 2137 |
| 80 | Accept | 9.3839 | 1.2513 | 8.8377 | 161 | ensemble | Method: LSBoost |
| | | | | | | | NumLearningCycles: 225 |
| | | | | | | | MinLeafSize: 4427 |
|=============================================================================================================================================|
| Iter | Eval | log(1+valLoss)| Time for training | Observed min | Training set | Learner | Hyperparameter: Value |
| | result | | & validation (sec)| validation loss | size | | |
|=============================================================================================================================================|
| 81 | Accept | 9.3187 | 0.065288 | 8.8377 | 161 | tree | MinLeafSize: 1 |
| 82 | Accept | 73.182 | 0.65686 | 8.8377 | 161 | svm | BoxConstraint: 8.1923 |
| | | | | | | | KernelScale: 49.754 |
| | | | | | | | Epsilon: 26.414 |
| 83 | Accept | 8.9351 | 0.062065 | 8.8377 | 643 | tree | MinLeafSize: 65 |
| 84 | Accept | 8.8466 | 3.9785 | 8.8377 | 2569 | ensemble | Method: Bag |
| | | | | | | | NumLearningCycles: 281 |
| | | | | | | | MinLeafSize: 4 |
| 85 | Best | 8.8186 | 4.6234 | 8.8186 | 10276 | ensemble | Method: Bag |
| | | | | | | | NumLearningCycles: 208 |
| | | | | | | | MinLeafSize: 16 |
| 86 | Accept | 49.608 | 4.8881 | 8.8186 | 161 | svm | BoxConstraint: 0.0038653 |
| | | | | | | | KernelScale: 0.084163 |
| | | | | | | | Epsilon: 0.17521 |
| 87 | Accept | 9.3834 | 0.07619 | 8.8186 | 161 | tree | MinLeafSize: 4107 |
| 88 | Accept | 26.492 | 0.67516 | 8.8186 | 161 | svm | BoxConstraint: 2.5636 |
| | | | | | | | KernelScale: 26.944 |
| | | | | | | | Epsilon: 6.7933 |
| 89 | Accept | 9.3862 | 0.058577 | 8.8186 | 161 | svm | BoxConstraint: 0.0046431 |
| | | | | | | | KernelScale: 0.0018285 |
| | | | | | | | Epsilon: 912.14 |
| 90 | Accept | 9.3978 | 0.1019 | 8.8186 | 643 | tree | MinLeafSize: 1 |
| 91 | Accept | 9.183 | 0.059411 | 8.8186 | 161 | tree | MinLeafSize: 7 |
| 92 | Accept | 9.4018 | 0.065758 | 8.8186 | 161 | svm | BoxConstraint: 0.011254 |
| | | | | | | | KernelScale: 1.6707 |
| | | | | | | | Epsilon: 1282.9 |
| 93 | Accept | 9.4118 | 1.3933 | 8.8186 | 161 | ensemble | Method: LSBoost |
| | | | | | | | NumLearningCycles: 246 |
| | | | | | | | MinLeafSize: 5704 |
| 94 | Accept | 50.857 | 4.4175 | 8.8186 | 161 | svm | BoxConstraint: 184.91 |
| | | | | | | | KernelScale: 300 |
| | | | | | | | Epsilon: 9.9176 |
| 95 | Accept | 9.2085 | 0.087503 | 8.8186 | 643 | tree | MinLeafSize: 7 |
| 96 | Accept | 9.4498 | 4.023 | 8.8186 | 161 | svm | BoxConstraint: 0.0021245 |
| | | | | | | | KernelScale: 103.57 |
| | | | | | | | Epsilon: 59.501 |
| 97 | Accept | 9.3829 | 0.0519 | 8.8186 | 161 | tree | MinLeafSize: 225 |
| 98 | Accept | 9.4148 | 0.64092 | 8.8186 | 161 | svm | BoxConstraint: 1.1581 |
| | | | | | | | KernelScale: 375.69 |
| | | | | | | | Epsilon: 1.2079 |
| 99 | Accept | 9.4039 | 0.057192 | 8.8186 | 161 | svm | BoxConstraint: 0.0046686 |
| | | | | | | | KernelScale: 0.0075742 |
| | | | | | | | Epsilon: 6458.5 |
| 100 | Accept | 9.2181 | 0.05773 | 8.8186 | 643 | tree | MinLeafSize: 225 |
|=============================================================================================================================================|
| Iter | Eval | log(1+valLoss)| Time for training | Observed min | Training set | Learner | Hyperparameter: Value |
| | result | | & validation (sec)| validation loss | size | | |
|=============================================================================================================================================|
| 101 | Accept | 9.3843 | 1.2718 | 8.8186 | 161 | ensemble | Method: Bag |
| | | | | | | | NumLearningCycles: 253 |
| | | | | | | | MinLeafSize: 544 |
| 102 | Accept | 9.3807 | 1.3051 | 8.8186 | 161 | ensemble | Method: LSBoost |
| | | | | | | | NumLearningCycles: 228 |
| | | | | | | | MinLeafSize: 100 |
| 103 | Accept | 21.2 | 0.66422 | 8.8186 | 161 | svm | BoxConstraint: 0.0091956 |
| | | | | | | | KernelScale: 6.027 |
| | | | | | | | Epsilon: 0.19667 |
| 104 | Accept | 10.175 | 0.079159 | 8.8186 | 161 | svm | BoxConstraint: 0.10113 |
| | | | | | | | KernelScale: 72.6 |
| | | | | | | | Epsilon: 70.924 |
| 105 | Accept | 8.9431 | 3.0941 | 8.8186 | 643 | ensemble | Method: LSBoost |
| | | | | | | | NumLearningCycles: 228 |
| | | | | | | | MinLeafSize: 100 |
| 106 | Accept | 8.8463 | 4.1395 | 8.8186 | 2569 | ensemble | Method: Bag |
| | | | | | | | NumLearningCycles: 285 |
| | | | | | | | MinLeafSize: 12 |
| 107 | Accept | 12.042 | 4.2272 | 8.8186 | 161 | svm | BoxConstraint: 0.0062352 |
| | | | | | | | KernelScale: 0.1105 |
| | | | | | | | Epsilon: 0.54085 |
| 108 | Accept | 9.3848 | 1.2747 | 8.8186 | 161 | ensemble | Method: LSBoost |
| | | | | | | | NumLearningCycles: 218 |
| | | | | | | | MinLeafSize: 840 |
| 109 | Accept | 9.3934 | 0.058925 | 8.8186 | 161 | svm | BoxConstraint: 5.6969 |
| | | | | | | | KernelScale: 0.023262 |
| | | | | | | | Epsilon: 7846.5 |
| 110 | Accept | 9.0115 | 0.073708 | 8.8186 | 161 | tree | MinLeafSize: 37 |
| 111 | Accept | 8.9938 | 0.060299 | 8.8186 | 643 | tree | MinLeafSize: 37 |
| 112 | Accept | 9.391 | 0.048814 | 8.8186 | 161 | tree | MinLeafSize: 1820 |
| 113 | Accept | 9.3873 | 0.074416 | 8.8186 | 161 | svm | BoxConstraint: 10.972 |
| | | | | | | | KernelScale: 0.0019127 |
| | | | | | | | Epsilon: 2.2406 |
| 114 | Accept | 8.9535 | 1.9056 | 8.8186 | 161 | ensemble | Method: Bag |
| | | | | | | | NumLearningCycles: 266 |
| | | | | | | | MinLeafSize: 16 |
| 115 | Accept | 9.397 | 1.0038 | 8.8186 | 161 | ensemble | Method: Bag |
| | | | | | | | NumLearningCycles: 201 |
| | | | | | | | MinLeafSize: 474 |
| 116 | Accept | 8.8859 | 2.4423 | 8.8186 | 643 | ensemble | Method: Bag |
| | | | | | | | NumLearningCycles: 266 |
| | | | | | | | MinLeafSize: 16 |
| 117 | Accept | 9.3736 | 0.062757 | 8.8186 | 161 | tree | MinLeafSize: 4 |
| 118 | Accept | 9.3833 | 1.6432 | 8.8186 | 161 | ensemble | Method: LSBoost |
| | | | | | | | NumLearningCycles: 286 |
| | | | | | | | MinLeafSize: 415 |
| 119 | Accept | 9.4034 | 0.052013 | 8.8186 | 161 | tree | MinLeafSize: 163 |
| 120 | Accept | 65.505 | 4.21 | 8.8186 | 161 | svm | BoxConstraint: 126.42 |
| | | | | | | | KernelScale: 0.00956 |
| | | | | | | | Epsilon: 0.77659 |
|=============================================================================================================================================|
| Iter | Eval | log(1+valLoss)| Time for training | Observed min | Training set | Learner | Hyperparameter: Value |
| | result | | & validation (sec)| validation loss | size | | |
|=============================================================================================================================================|
| 121 | Accept | 9.3102 | 0.098523 | 8.8186 | 643 | tree | MinLeafSize: 4 |
| 122 | Accept | 15.235 | 3.8075 | 8.8186 | 161 | svm | BoxConstraint: 0.042479 |
| | | | | | | | KernelScale: 0.054739 |
| | | | | | | | Epsilon: 126.07 |
| 123 | Accept | 9.3993 | 1.2692 | 8.8186 | 161 | ensemble | Method: LSBoost |
| | | | | | | | NumLearningCycles: 220 |
| | | | | | | | MinLeafSize: 305 |
| 124 | Accept | 9.3933 | 0.047521 | 8.8186 | 161 | tree | MinLeafSize: 181 |
| 125 | Accept | 9.4005 | 0.064158 | 8.8186 | 161 | svm | BoxConstraint: 0.75184 |
| | | | | | | | KernelScale: 103.16 |
| | | | | | | | Epsilon: 7561.9 |
| 126 | Accept | 9.3843 | 1.5854 | 8.8186 | 643 | ensemble | Method: LSBoost |
| | | | | | | | NumLearningCycles: 286 |
| | | | | | | | MinLeafSize: 415 |
| 127 | Accept | 8.8356 | 3.6654 | 8.8186 | 2569 | ensemble | Method: Bag |
| | | | | | | | NumLearningCycles: 266 |
| | | | | | | | MinLeafSize: 16 |
| 128 | Accept | 9.3867 | 0.063994 | 8.8186 | 161 | tree | MinLeafSize: 5651 |
| 129 | Accept | 13.063 | 2.1824 | 8.8186 | 161 | svm | BoxConstraint: 42.956 |
| | | | | | | | KernelScale: 0.15102 |
| | | | | | | | Epsilon: 150.18 |
| 130 | Accept | 9.6326 | 0.60577 | 8.8186 | 161 | svm | BoxConstraint: 0.001179 |
| | | | | | | | KernelScale: 210.03 |
| | | | | | | | Epsilon: 0.64499 |
| 131 | Accept | 9.3849 | 1.0936 | 8.8186 | 161 | ensemble | Method: Bag |
| | | | | | | | NumLearningCycles: 207 |
| | | | | | | | MinLeafSize: 3437 |
| 132 | Accept | 9.3808 | 0.0571 | 8.8186 | 643 | tree | MinLeafSize: 4107 |
| 133 | Accept | 36.663 | 0.084854 | 8.8186 | 161 | svm | BoxConstraint: 0.0013618 |
| | | | | | | | KernelScale: 5.0765 |
| | | | | | | | Epsilon: 127.19 |
| 134 | Accept | 16.268 | 3.842 | 8.8186 | 161 | svm | BoxConstraint: 0.0064316 |
| | | | | | | | KernelScale: 0.19009 |
| | | | | | | | Epsilon: 1.1912 |
| 135 | Accept | 9.5749 | 0.6606 | 8.8186 | 161 | svm | BoxConstraint: 0.089516 |
| | | | | | | | KernelScale: 127.63 |
| | | | | | | | Epsilon: 1.7522 |
| 136 | Accept | 9.3917 | 1.1714 | 8.8186 | 161 | ensemble | Method: Bag |
| | | | | | | | NumLearningCycles: 234 |
| | | | | | | | MinLeafSize: 5148 |
| 137 | Accept | 8.9512 | 2.9923 | 8.8186 | 643 | ensemble | Method: LSBoost |
| | | | | | | | NumLearningCycles: 269 |
| | | | | | | | MinLeafSize: 101 |
| 138 | Accept | 9.1052 | 0.0628 | 8.8186 | 161 | tree | MinLeafSize: 7 |
| 139 | Accept | 9.398 | 0.081349 | 8.8186 | 161 | svm | BoxConstraint: 0.016058 |
| | | | | | | | KernelScale: 183.58 |
| | | | | | | | Epsilon: 503.13 |
| 140 | Accept | 9.4164 | 0.056624 | 8.8186 | 161 | tree | MinLeafSize: 1758 |
|=============================================================================================================================================|
| Iter | Eval | log(1+valLoss)| Time for training | Observed min | Training set | Learner | Hyperparameter: Value |
| | result | | & validation (sec)| validation loss | size | | |
|=============================================================================================================================================|
| 141 | Accept | 9.4052 | 0.061562 | 8.8186 | 161 | svm | BoxConstraint: 0.023222 |
| | | | | | | | KernelScale: 76.906 |
| | | | | | | | Epsilon: 8814 |
| 142 | Accept | 9.1784 | 0.082115 | 8.8186 | 643 | tree | MinLeafSize: 7 |
| 143 | Accept | 9.2477 | 2.0155 | 8.8186 | 161 | ensemble | Method: LSBoost |
| | | | | | | | NumLearningCycles: 272 |
| | | | | | | | MinLeafSize: 1 |
| 144 | Accept | 9.4018 | 0.06487 | 8.8186 | 161 | svm | BoxConstraint: 626.86 |
| | | | | | | | KernelScale: 0.43541 |
| | | | | | | | Epsilon: 1627.4 |
| 145 | Accept | 9.3975 | 0.058275 | 8.8186 | 161 | svm | BoxConstraint: 0.0028588 |
| | | | | | | | KernelScale: 209.66 |
| | | | | | | | Epsilon: 4232.3 |
| 146 | Accept | 9.521 | 0.6356 | 8.8186 | 161 | svm | BoxConstraint: 0.083407 |
| | | | | | | | KernelScale: 312.85 |
| | | | | | | | Epsilon: 0.20668 |
| 147 | Accept | 8.9708 | 3.2479 | 8.8186 | 643 | ensemble | Method: LSBoost |
| | | | | | | | NumLearningCycles: 272 |
| | | | | | | | MinLeafSize: 1 |
| 148 | Accept | 8.9616 | 0.10093 | 8.8186 | 2569 | tree | MinLeafSize: 34 |
| 149 | Accept | 15.713 | 4.9592 | 8.8186 | 161 | svm | BoxConstraint: 0.019721 |
| | | | | | | | KernelScale: 0.006631 |
| | | | | | | | Epsilon: 0.81317 |
| 150 | Accept | 61.246 | 2.2315 | 8.8186 | 161 | svm | BoxConstraint: 0.10628 |
| | | | | | | | KernelScale: 0.26584 |
| | | | | | | | Epsilon: 56.177 |
| 151 | Accept | 9.3827 | 1.118 | 8.8186 | 161 | ensemble | Method: Bag |
| | | | | | | | NumLearningCycles: 214 |
| | | | | | | | MinLeafSize: 314 |
| 152 | Accept | 9.776 | 4.5082 | 8.8186 | 161 | svm | BoxConstraint: 0.0013601 |
| | | | | | | | KernelScale: 0.046336 |
| | | | | | | | Epsilon: 5.0766 |
| 153 | Accept | 9.3125 | 1.2559 | 8.8186 | 643 | ensemble | Method: Bag |
| | | | | | | | NumLearningCycles: 214 |
| | | | | | | | MinLeafSize: 314 |
| 154 | Accept | 9.397 | 1.4413 | 8.8186 | 161 | ensemble | Method: Bag |
| | | | | | | | NumLearningCycles: 273 |
| | | | | | | | MinLeafSize: 594 |
| 155 | Accept | 9.3904 | 0.067118 | 8.8186 | 161 | svm | BoxConstraint: 0.0014004 |
| | | | | | | | KernelScale: 41.954 |
| | | | | | | | Epsilon: 6132.6 |
| 156 | Accept | 11.159 | 0.074313 | 8.8186 | 161 | svm | BoxConstraint: 0.013397 |
| | | | | | | | KernelScale: 9.1715 |
| | | | | | | | Epsilon: 81.019 |
| 157 | Accept | 22.357 | 4.3335 | 8.8186 | 161 | svm | BoxConstraint: 0.41907 |
| | | | | | | | KernelScale: 0.010689 |
| | | | | | | | Epsilon: 13.091 |
| 158 | Accept | 9.3881 | 1.2611 | 8.8186 | 643 | ensemble | Method: LSBoost |
| | | | | | | | NumLearningCycles: 225 |
| | | | | | | | MinLeafSize: 4427 |
| 159 | Accept | 9.4028 | 0.067058 | 8.8186 | 161 | svm | BoxConstraint: 0.036022 |
| | | | | | | | KernelScale: 8.618 |
| | | | | | | | Epsilon: 12523 |
| 160 | Accept | 9.5619 | 4.8535 | 8.8186 | 161 | svm | BoxConstraint: 5.6235 |
| | | | | | | | KernelScale: 0.020708 |
| | | | | | | | Epsilon: 0.15719 |
|=============================================================================================================================================|
| Iter | Eval | log(1+valLoss)| Time for training | Observed min | Training set | Learner | Hyperparameter: Value |
| | result | | & validation (sec)| validation loss | size | | |
|=============================================================================================================================================|
| 161 | Accept | 9.385 | 0.070467 | 8.8186 | 161 | tree | MinLeafSize: 2083 |
| 162 | Accept | 9.4042 | 0.061121 | 8.8186 | 161 | svm | BoxConstraint: 212.83 |
| | | | | | | | KernelScale: 0.0011315 |
| | | | | | | | Epsilon: 4.8239 |
| 163 | Accept | 9.3832 | 1.3395 | 8.8186 | 643 | ensemble | Method: Bag |
| | | | | | | | NumLearningCycles: 253 |
| | | | | | | | MinLeafSize: 544 |
| 164 | Accept | 9.4427 | 0.062918 | 8.8186 | 161 | svm | BoxConstraint: 40.982 |
| | | | | | | | KernelScale: 51.518 |
| | | | | | | | Epsilon: 276.22 |
| 165 | Accept | 9.3838 | 0.052175 | 8.8186 | 161 | tree | MinLeafSize: 259 |
| 166 | Accept | 9.3923 | 0.044845 | 8.8186 | 161 | tree | MinLeafSize: 174 |
| 167 | Accept | 9.3843 | 0.064853 | 8.8186 | 161 | svm | BoxConstraint: 2.4613 |
| | | | | | | | KernelScale: 0.0059067 |
| | | | | | | | Epsilon: 2318.5 |
| 168 | Accept | 9.2331 | 0.058123 | 8.8186 | 643 | tree | MinLeafSize: 259 |
| 169 | Accept | 8.9465 | 0.09373 | 8.8186 | 2569 | tree | MinLeafSize: 33 |
| 170 | Accept | 8.8205 | 5.9209 | 8.8186 | 10276 | ensemble | Method: Bag |
| | | | | | | | NumLearningCycles: 266 |
| | | | | | | | MinLeafSize: 16 |
| 171 | Accept | 9.0308 | 0.055452 | 8.8186 | 161 | tree | MinLeafSize: 25 |
| 172 | Accept | 9.4106 | 0.064019 | 8.8186 | 161 | svm | BoxConstraint: 5.1299 |
| | | | | | | | KernelScale: 0.0049434 |
| | | | | | | | Epsilon: 2964.7 |
| 173 | Accept | 8.9875 | 0.049886 | 8.8186 | 161 | tree | MinLeafSize: 17 |
| 174 | Accept | 9.6815 | 0.068647 | 8.8186 | 161 | svm | BoxConstraint: 0.012521 |
| | | | | | | | KernelScale: 5.8218 |
| | | | | | | | Epsilon: 158.28 |
| 175 | Accept | 9.0889 | 0.080584 | 8.8186 | 643 | tree | MinLeafSize: 17 |
| 176 | Accept | 9.0743 | 0.051929 | 8.8186 | 161 | tree | MinLeafSize: 9 |
| 177 | Accept | 50.143 | 0.53578 | 8.8186 | 161 | svm | BoxConstraint: 0.0025675 |
| | | | | | | | KernelScale: 2.9123 |
| | | | | | | | Epsilon: 2.7823 |
| 178 | Accept | 11.317 | 0.65696 | 8.8186 | 161 | svm | BoxConstraint: 0.0013653 |
| | | | | | | | KernelScale: 0.72963 |
| | | | | | | | Epsilon: 1.9059 |
| 179 | Accept | 8.9881 | 1.9317 | 8.8186 | 161 | ensemble | Method: Bag |
| | | | | | | | NumLearningCycles: 273 |
| | | | | | | | MinLeafSize: 4 |
| 180 | Accept | 8.8611 | 2.3584 | 8.8186 | 643 | ensemble | Method: Bag |
| | | | | | | | NumLearningCycles: 273 |
| | | | | | | | MinLeafSize: 4 |
|=============================================================================================================================================|
| Iter | Eval | log(1+valLoss)| Time for training | Observed min | Training set | Learner | Hyperparameter: Value |
| | result | | & validation (sec)| validation loss | size | | |
|=============================================================================================================================================|
| 181 | Accept | 17.128 | 0.082904 | 8.8186 | 161 | svm | BoxConstraint: 882.02 |
| | | | | | | | KernelScale: 3.6447 |
| | | | | | | | Epsilon: 40.81 |
| 182 | Accept | 9.3873 | 0.059449 | 8.8186 | 161 | svm | BoxConstraint: 0.036152 |
| | | | | | | | KernelScale: 128.56 |
| | | | | | | | Epsilon: 676.9 |
| 183 | Accept | 14.295 | 0.59637 | 8.8186 | 161 | svm | BoxConstraint: 0.036148 |
| | | | | | | | KernelScale: 5.6466 |
| | | | | | | | Epsilon: 3.4635 |
| 184 | Accept | 9.3841 | 1.4813 | 8.8186 | 161 | ensemble | Method: Bag |
| | | | | | | | NumLearningCycles: 299 |
| | | | | | | | MinLeafSize: 1158 |
| 185 | Accept | 8.9781 | 0.071583 | 8.8186 | 643 | tree | MinLeafSize: 25 |
| 186 | Accept | 9.4077 | 0.06309 | 8.8186 | 161 | svm | BoxConstraint: 349.21 |
| | | | | | | | KernelScale: 0.042446 |
| | | | | | | | Epsilon: 9446.5 |
| 187 | Accept | 63.652 | 0.51835 | 8.8186 | 161 | svm | BoxConstraint: 55.367 |
| | | | | | | | KernelScale: 2.9867 |
| | | | | | | | Epsilon: 0.37288 |
| 188 | Accept | 9.4193 | 0.057529 | 8.8186 | 161 | svm | BoxConstraint: 22.899 |
| | | | | | | | KernelScale: 0.0048942 |
| | | | | | | | Epsilon: 483.9 |
| 189 | Accept | 36.23 | 0.50743 | 8.8186 | 161 | svm | BoxConstraint: 0.5866 |
| | | | | | | | KernelScale: 9.2803 |
| | | | | | | | Epsilon: 21.876 |
| 190 | Accept | 9.1316 | 0.079127 | 8.8186 | 643 | tree | MinLeafSize: 9 |
| 191 | Accept | 8.84 | 3.7635 | 8.8186 | 2569 | ensemble | Method: Bag |
| | | | | | | | NumLearningCycles: 273 |
| | | | | | | | MinLeafSize: 4 |
| 192 | Accept | 9.3821 | 1.563 | 8.8186 | 161 | ensemble | Method: LSBoost |
| | | | | | | | NumLearningCycles: 286 |
| | | | | | | | MinLeafSize: 584 |
| 193 | Accept | 8.9676 | 1.8838 | 8.8186 | 161 | ensemble | Method: LSBoost |
| | | | | | | | NumLearningCycles: 267 |
| | | | | | | | MinLeafSize: 19 |
| 194 | Accept | 9.1405 | 0.069161 | 8.8186 | 161 | tree | MinLeafSize: 7 |
| 195 | Accept | 9.4212 | 0.09857 | 8.8186 | 161 | svm | BoxConstraint: 50.571 |
| | | | | | | | KernelScale: 0.024255 |
| | | | | | | | Epsilon: 7431.5 |
| 196 | Accept | 8.9856 | 3.4297 | 8.8186 | 643 | ensemble | Method: LSBoost |
| | | | | | | | NumLearningCycles: 267 |
| | | | | | | | MinLeafSize: 19 |
| 197 | Accept | 9.0698 | 1.7118 | 8.8186 | 161 | ensemble | Method: LSBoost |
| | | | | | | | NumLearningCycles: 237 |
| | | | | | | | MinLeafSize: 3 |
| 198 | Accept | 9.3841 | 1.1616 | 8.8186 | 161 | ensemble | Method: Bag |
| | | | | | | | NumLearningCycles: 219 |
| | | | | | | | MinLeafSize: 135 |
| 199 | Accept | 9.3855 | 1.2281 | 8.8186 | 161 | ensemble | Method: LSBoost |
| | | | | | | | NumLearningCycles: 220 |
| | | | | | | | MinLeafSize: 1640 |
| 200 | Accept | 9.3889 | 0.066239 | 8.8186 | 161 | svm | BoxConstraint: 0.79242 |
| | | | | | | | KernelScale: 0.02442 |
| | | | | | | | Epsilon: 3825.6 |
|=============================================================================================================================================|
| Iter | Eval | log(1+valLoss)| Time for training | Observed min | Training set | Learner | Hyperparameter: Value |
| | result | | & validation (sec)| validation loss | size | | |
|=============================================================================================================================================|
| 201 | Accept | 8.9793 | 3.1501 | 8.8186 | 643 | ensemble | Method: LSBoost |
| | | | | | | | NumLearningCycles: 237 |
| | | | | | | | MinLeafSize: 3 |
| 202 | Accept | 9.4226 | 0.058343 | 8.8186 | 161 | svm | BoxConstraint: 0.0095052 |
| | | | | | | | KernelScale: 41.559 |
| | | | | | | | Epsilon: 1783.5 |
| 203 | Accept | 10.347 | 4.2165 | 8.8186 | 161 | svm | BoxConstraint: 131.23 |
| | | | | | | | KernelScale: 0.072051 |
| | | | | | | | Epsilon: 7.455 |
| 204 | Accept | 9.3921 | 0.065431 | 8.8186 | 161 | tree | MinLeafSize: 2331 |
| 205 | Accept | 9.3958 | 0.066415 | 8.8186 | 161 | svm | BoxConstraint: 0.0016799 |
| | | | | | | | KernelScale: 425.35 |
| | | | | | | | Epsilon: 258.47 |
| 206 | Accept | 9.1869 | 0.07482 | 8.8186 | 643 | tree | MinLeafSize: 7 |
| 207 | Accept | 9.3103 | 0.046643 | 8.8186 | 161 | tree | MinLeafSize: 58 |
| 208 | Accept | 9.3878 | 0.04433 | 8.8186 | 161 | tree | MinLeafSize: 1330 |
| 209 | Accept | 9.4127 | 0.062485 | 8.8186 | 161 | svm | BoxConstraint: 0.33434 |
| | | | | | | | KernelScale: 0.015733 |
| | | | | | | | Epsilon: 2799.4 |
| 210 | Accept | 36.153 | 0.62403 | 8.8186 | 161 | svm | BoxConstraint: 0.1378 |
| | | | | | | | KernelScale: 7.1397 |
| | | | | | | | Epsilon: 15.041 |
| 211 | Accept | 8.9388 | 0.059248 | 8.8186 | 643 | tree | MinLeafSize: 58 |
| 212 | Accept | 8.9134 | 0.090664 | 8.8186 | 2569 | tree | MinLeafSize: 65 |
| 213 | Accept | 9.3964 | 0.061733 | 8.8186 | 161 | svm | BoxConstraint: 0.26343 |
| | | | | | | | KernelScale: 0.00887 |
| | | | | | | | Epsilon: 3917.2 |
| 214 | Accept | 9.3912 | 0.0468 | 8.8186 | 161 | tree | MinLeafSize: 2438 |
| 215 | Accept | 12.36 | 0.56796 | 8.8186 | 161 | svm | BoxConstraint: 577.35 |
| | | | | | | | KernelScale: 30.71 |
| | | | | | | | Epsilon: 1.0514 |
| 216 | Accept | 9.1224 | 1.7567 | 8.8186 | 161 | ensemble | Method: LSBoost |
| | | | | | | | NumLearningCycles: 249 |
| | | | | | | | MinLeafSize: 44 |
| 217 | Accept | 9.0025 | 3.6564 | 8.8186 | 643 | ensemble | Method: LSBoost |
| | | | | | | | NumLearningCycles: 249 |
| | | | | | | | MinLeafSize: 44 |
| 218 | Accept | 9.3834 | 1.1499 | 8.8186 | 161 | ensemble | Method: Bag |
| | | | | | | | NumLearningCycles: 228 |
| | | | | | | | MinLeafSize: 102 |
| 219 | Accept | 9.028 | 2.0525 | 8.8186 | 161 | ensemble | Method: LSBoost |
| | | | | | | | NumLearningCycles: 253 |
| | | | | | | | MinLeafSize: 2 |
| 220 | Accept | 9.3824 | 0.060217 | 8.8186 | 161 | tree | MinLeafSize: 374 |
|=============================================================================================================================================|
| Iter | Eval | log(1+valLoss)| Time for training | Observed min | Training set | Learner | Hyperparameter: Value |
| | result | | & validation (sec)| validation loss | size | | |
|=============================================================================================================================================|
| 221 | Accept | 9.3911 | 0.085777 | 8.8186 | 161 | svm | BoxConstraint: 12.507 |
| | | | | | | | KernelScale: 0.012484 |
| | | | | | | | Epsilon: 227.96 |
| 222 | Accept | 8.9964 | 3.5015 | 8.8186 | 643 | ensemble | Method: LSBoost |
| | | | | | | | NumLearningCycles: 253 |
| | | | | | | | MinLeafSize: 2 |
| 223 | Accept | 9.1692 | 0.06141 | 8.8186 | 161 | tree | MinLeafSize: 9 |
| 224 | Accept | 54.023 | 0.53103 | 8.8186 | 161 | svm | BoxConstraint: 402.26 |
| | | | | | | | KernelScale: 23.129 |
| | | | | | | | Epsilon: 0.15314 |
| 225 | Accept | 9.3834 | 0.061383 | 8.8186 | 161 | tree | MinLeafSize: 1 |
| 226 | Accept | 8.9297 | 0.050965 | 8.8186 | 161 | tree | MinLeafSize: 30 |
| 227 | Accept | 8.9426 | 0.069941 | 8.8186 | 643 | tree | MinLeafSize: 30 |
| 228 | Accept | 9.3909 | 1.2347 | 8.8186 | 161 | ensemble | Method: Bag |
| | | | | | | | NumLearningCycles: 242 |
| | | | | | | | MinLeafSize: 193 |
| 229 | Accept | 14.093 | 0.51359 | 8.8186 | 161 | svm | BoxConstraint: 2.7008 |
| | | | | | | | KernelScale: 8.988 |
| | | | | | | | Epsilon: 0.31364 |
| 230 | Accept | 8.9475 | 1.8933 | 8.8186 | 161 | ensemble | Method: Bag |
| | | | | | | | NumLearningCycles: 274 |
| | | | | | | | MinLeafSize: 2 |
| 231 | Accept | 9.3847 | 0.060031 | 8.8186 | 161 | tree | MinLeafSize: 5326 |
| 232 | Accept | 8.8871 | 2.3958 | 8.8186 | 643 | ensemble | Method: Bag |
| | | | | | | | NumLearningCycles: 274 |
| | | | | | | | MinLeafSize: 2 |
| 233 | Accept | 8.8526 | 3.8394 | 8.8186 | 2569 | ensemble | Method: Bag |
| | | | | | | | NumLearningCycles: 274 |
| | | | | | | | MinLeafSize: 2 |
| 234 | Accept | 9.6167 | 0.71275 | 8.8186 | 161 | svm | BoxConstraint: 0.0033201 |
| | | | | | | | KernelScale: 11.038 |
| | | | | | | | Epsilon: 6.2594 |
| 235 | Accept | 9.3917 | 0.056137 | 8.8186 | 161 | tree | MinLeafSize: 114 |
| 236 | Accept | 45.36 | 4.8199 | 8.8186 | 161 | svm | BoxConstraint: 947.1 |
| | | | | | | | KernelScale: 0.01755 |
| | | | | | | | Epsilon: 38.99 |
| 237 | Accept | 32.375 | 0.43733 | 8.8186 | 161 | svm | BoxConstraint: 80.29 |
| | | | | | | | KernelScale: 131.32 |
| | | | | | | | Epsilon: 1.4516 |
| 238 | Accept | 9.1149 | 0.072948 | 8.8186 | 643 | tree | MinLeafSize: 9 |
| 239 | Accept | 9.3992 | 0.058396 | 8.8186 | 161 | svm | BoxConstraint: 0.0087101 |
| | | | | | | | KernelScale: 0.049442 |
| | | | | | | | Epsilon: 3014.3 |
| 240 | Accept | 32.828 | 0.68213 | 8.8186 | 161 | svm | BoxConstraint: 0.01464 |
| | | | | | | | KernelScale: 30.001 |
| | | | | | | | Epsilon: 34.092 |
|=============================================================================================================================================|
| Iter | Eval | log(1+valLoss)| Time for training | Observed min | Training set | Learner | Hyperparameter: Value |
| | result | | & validation (sec)| validation loss | size | | |
|=============================================================================================================================================|
| 241 | Accept | 76.162 | 5.2571 | 8.8186 | 161 | svm | BoxConstraint: 25.679 |
| | | | | | | | KernelScale: 0.058947 |
| | | | | | | | Epsilon: 5.5863 |
| 242 | Accept | 9.0454 | 2.2506 | 8.8186 | 161 | ensemble | Method: LSBoost |
| | | | | | | | NumLearningCycles: 275 |
| | | | | | | | MinLeafSize: 2 |
| 243 | Accept | 9.0188 | 4.1799 | 8.8186 | 643 | ensemble | Method: LSBoost |
| | | | | | | | NumLearningCycles: 275 |
| | | | | | | | MinLeafSize: 2 |
| 244 | Accept | 9.3987 | 0.071851 | 8.8186 | 161 | svm | BoxConstraint: 345.64 |
| | | | | | | | KernelScale: 0.90102 |
| | | | | | | | Epsilon: 370.38 |
| 245 | Accept | 49.943 | 0.60096 | 8.8186 | 161 | svm | BoxConstraint: 391.91 |
| | | | | | | | KernelScale: 3.856 |
| | | | | | | | Epsilon: 12.255 |
| 246 | Accept | 9.4879 | 0.084173 | 8.8186 | 161 | tree | MinLeafSize: 2 |
| 247 | Accept | 9.3865 | 1.404 | 8.8186 | 161 | ensemble | Method: Bag |
| | | | | | | | NumLearningCycles: 263 |
| | | | | | | | MinLeafSize: 255 |
| 248 | Accept | 9.3816 | 1.6134 | 8.8186 | 643 | ensemble | Method: LSBoost |
| | | | | | | | NumLearningCycles: 286 |
| | | | | | | | MinLeafSize: 584 |
| 249 | Accept | 45.435 | 0.10098 | 8.8186 | 161 | svm | BoxConstraint: 0.005269 |
| | | | | | | | KernelScale: 0.0040109 |
| | | | | | | | Epsilon: 86.961 |
| 250 | Accept | 9.3853 | 1.4575 | 8.8186 | 161 | ensemble | Method: Bag |
| | | | | | | | NumLearningCycles: 280 |
| | | | | | | | MinLeafSize: 290 |
| 251 | Accept | 9.6044 | 0.69076 | 8.8186 | 161 | svm | BoxConstraint: 291.8 |
| | | | | | | | KernelScale: 755.95 |
| | | | | | | | Epsilon: 1.3387 |
| 252 | Accept | 9.1305 | 2.1799 | 8.8186 | 161 | ensemble | Method: Bag |
| | | | | | | | NumLearningCycles: 296 |
| | | | | | | | MinLeafSize: 7 |
| 253 | Accept | 8.8709 | 2.5698 | 8.8186 | 643 | ensemble | Method: Bag |
| | | | | | | | NumLearningCycles: 296 |
| | | | | | | | MinLeafSize: 7 |
| 254 | Accept | 8.8373 | 3.8823 | 8.8186 | 2569 | ensemble | Method: Bag |
| | | | | | | | NumLearningCycles: 296 |
| | | | | | | | MinLeafSize: 7 |
| 255 | Accept | 8.8187 | 6.4895 | 8.8186 | 10276 | ensemble | Method: Bag |
| | | | | | | | NumLearningCycles: 296 |
| | | | | | | | MinLeafSize: 7 |__________________________________________________________
Optimization completed.
Total iterations: 255
Total elapsed time: 348.5896 seconds
Total time for training and validation: 307.7084 secondsBest observed learner is an ensemble model with:
Learner: ensemble
Method: Bag
NumLearningCycles: 208
MinLeafSize: 16
Observed log(1 + valLoss): 8.8186
Time for training and validation: 4.6234 secondsDocumentation for fitrauto display

PredictorNames: {1×30 cell}
ResponseName: ‘metastatic_diagnosis_period’
CategoricalPredictors: [1 2]
ResponseTransform: ‘none’
NumTrained: 208Properties, Methods
Create Submission
Set ‘VariableNamingRule’ to ‘preserve’ to use the original column headers as table variable names.
patient_id | patient_race | payer_type | patient_state | patient_zip3 | Region | Division | patient_age | patient_gender | bmi | breast_cancer_diagnosis_code | breast_cancer_diagnosis_desc | metastatic_cancer_diagnosis_code | metastatic_first_novel_treatment | metastatic_first_novel_treatment_type | population | density | age_median | age_under_10 | age_10_to_19 | age_20s | age_30s | age_40s | age_50s | age_60s | age_70s | age_over_80 | male | female | married | |
---|---|---|---|---|---|---|---|---|---|---|---|---|---|---|---|---|---|---|---|---|---|---|---|---|---|---|---|---|---|---|
1 | 730681 | ” | ‘COMMERCIAL’ | ‘LA’ | 713 | ‘South’ | ‘West South Central’ | 55 | ‘F’ | NaN | ‘1746’ | ‘Malignant neoplasm of axillary tail of female breast’ | ‘C7981’ | NaN | NaN | 4.6391e+03 | 72.6643 | 41.5000 | 11.3952 | 13.4357 | 11.4214 | 11.4452 | 12.5619 | 13.0786 | 14.2571 | 7.7071 | 4.7286 | 50.0191 | 49.9809 | 42.3738 |
2 | 334212 | ‘Black’ | ” | ‘NC’ | 283 | ‘South’ | ‘South Atlantic’ | 60 | ‘F’ | 40 | ‘C50912’ | ‘Malignant neoplasm of unspecified site of left female breast’ | ‘C773’ | NaN | NaN | 1.0875e+04 | 217.9104 | 39.6447 | 11.2329 | 13.7158 | 15.0053 | 12.0158 | 11.5803 | 11.7711 | 12.7684 | 8.5184 | 3.4066 | 51.3263 | 48.6737 | 44.1355 |
3 | 571362 | ” | ‘COMMERCIAL’ | ‘TX’ | 794 | ‘South’ | ‘West South Central’ | 54 | ‘F’ | 32.3300 | ‘1742’ | ‘Malignant neoplasm of upper-inner quadrant of female breast’ | ‘C773’ | NaN | NaN | 1.8717e+04 | 1.0195e+03 | 30.3714 | 11 | 18.8643 | 23.1143 | 12.2429 | 9.8786 | 9.1214 | 8.3786 | 4.7786 | 2.6214 | 50.2857 | 49.7143 | 35.9857 |
4 | 907331 | ” | ‘COMMERCIAL’ | ‘TN’ | 373 | ‘South’ | ‘East South Central’ | 63 | ‘F’ | 27.0700 | ‘1748’ | ‘Malignant neoplasm of other specified sites of female breast’ | ‘C7951’ | NaN | NaN | 7.8048e+03 | 140.0545 | 44.3158 | 10.1947 | 12.6645 | 11.7026 | 10.5250 | 12.1329 | 14.9132 | 13.6816 | 9.8263 | 4.3632 | 49.4066 | 50.5934 | 52.2210 |
5 | 208382 | ‘Asian’ | ” | ‘WA’ | 980 | ‘West’ | ‘Pacific’ | 62 | ‘F’ | NaN | ‘C50411’ | ‘Malig neoplm of upper-outer quadrant of right female breast’ | ‘C787’ | NaN | NaN | 2.8628e+04 | 1.0918e+03 | 39.6793 | 12.1434 | 12.4623 | 11.3208 | 15.2132 | 14.4491 | 14.1057 | 11.2264 | 5.8415 | 3.2302 | 49.9698 | 50.0302 | 57.0962 |
6 | 852863 | ‘White’ | ‘MEDICARE ADVANTAGE’ | ‘CA’ | 914 | ‘West’ | ‘Pacific’ | 82 | ‘F’ | NaN | ‘1749’ | ‘Malignant neoplasm of breast (female), unspecified’ | ‘C7951’ | NaN | NaN | 3.9505e+04 | 4.0085e+03 | 37.5500 | 11.4875 | 11.4375 | 14.5125 | 16.6125 | 14.2875 | 13.6500 | 9.4750 | 5.3500 | 3.2250 | 49.6500 | 50.3500 | 43.3875 |
7 | 494644 | ‘Asian’ | ” | ‘MI’ | 483 | ‘Midwest’ | ‘East North Central’ | 67 | ‘F’ | 21.8000 | ‘C50911’ | ‘Malignant neoplasm of unsp site of right female breast’ | ‘C773’ | NaN | NaN | 2.0151e+04 | 724.9353 | 42.0784 | 11.0392 | 13.0098 | 11.6431 | 11.8882 | 13.0647 | 15.1098 | 12.8686 | 7.4000 | 3.9588 | 49.2922 | 50.7078 | 54.0137 |
8 | 852015 | ‘White’ | ‘MEDICAID’ | ‘FL’ | 336 | ‘South’ | ‘South Atlantic’ | 51 | ‘F’ | NaN | ‘C50919’ | ‘Malignant neoplasm of unsp site of unspecified female breast’ | ‘C7931’ | NaN | NaN | 3.0205e+04 | 1.5172e+03 | 35.6296 | 11.6963 | 14.2296 | 16.5926 | 15.2518 | 12.9037 | 11.6296 | 9.5259 | 5.4667 | 2.7444 | 49.6963 | 50.3037 | 39.3148 |
9 | 521061 | ‘Black’ | ‘MEDICAID’ | ‘CA’ | 917 | ‘West’ | ‘Pacific’ | 44 | ‘F’ | NaN | ‘C50011’ | ‘Malignant neoplasm of nipple and areola, right female breast’ | ‘C779’ | NaN | NaN | 4.3030e+04 | 2.0486e+03 | 38.8522 | 11.3065 | 12.8978 | 14.1217 | 13.5326 | 13.1609 | 13.3783 | 11.4739 | 6.3804 | 3.7370 | 49.0522 | 50.9478 | 48.5044 |
10 | 907023 | ‘White’ | ” | ‘PA’ | 160 | ‘Northeast’ | ‘Middle Atlantic’ | 70 | ‘F’ | NaN | ‘C50812’ | ‘Malignant neoplasm of ovrlp sites of left female breast’ | ‘C7951’ | NaN | NaN | 5.8126e+03 | 130.5714 | 44.6743 | 10.2943 | 12.1914 | 10.6971 | 11.6086 | 12.4543 | 14.5114 | 15.5171 | 8.0343 | 4.6629 | 50.5486 | 49.4514 | 56.5857 |
11 | 906063 | ” | ‘COMMERCIAL’ | ‘TX’ | 774 | ‘South’ | ‘West South Central’ | 27 | ‘F’ | 27.3700 | ‘C50912’ | ‘Malignant neoplasm of unspecified site of left female breast’ | ‘C773’ | NaN | NaN | 1.9403e+04 | 270.8549 | 39.1349 | 12.5188 | 15.7422 | 12.6547 | 13.3703 | 10.0297 | 12.6359 | 10.6875 | 7.1516 | 5.1937 | 50.0281 | 49.9719 | 51.7047 |
12 | 558053 | ‘Hispanic’ | ‘MEDICAID’ | ‘DE’ | 199 | ‘South’ | ‘South Atlantic’ | 44 | ‘F’ | 33.2000 | ‘C50112’ | ‘Malignant neoplasm of central portion of left female breast’ | ‘C773’ | NaN | NaN | 1.0754e+04 | 180.9974 | 45.9846 | 10.3359 | 11.5462 | 11.4692 | 10.6795 | 9.9436 | 14.4461 | 15.7180 | 10.7692 | 5.0949 | 50.7026 | 49.2974 | 50.9436 |
13 | 832804 | ‘White’ | ‘MEDICARE ADVANTAGE’ | ‘OH’ | 442 | ‘Midwest’ | ‘East North Central’ | 82 | ‘F’ | NaN | ‘19881’ | ‘Secondary malignant neoplasm of breast’ | ‘C7951’ | NaN | NaN | 13035 | 355.7023 | 42.8907 | 10.5953 | 14.0861 | 11.4395 | 11.4233 | 11.4302 | 14.9023 | 13.5488 | 8.5814 | 4.0023 | 49.9279 | 50.0721 | 54.1256 |
14 | 554976 | ” | ‘COMMERCIAL’ | ‘MT’ | 591 | ‘West’ | ‘Mountain’ | 71 | ‘F’ | 23.4800 | ‘1749’ | ‘Malignant neoplasm of breast (female), unspecified’ | ‘C773’ | NaN | NaN | 35549 | 367.6250 | 38.3250 | 13.0250 | 13.3000 | 12 | 14 | 12.6500 | 11.5500 | 12.5750 | 6.7500 | 4.1500 | 49.6000 | 50.4000 | 51.4750 |
⋮ |
patient_id | metastatic_diagnosis_period | |
---|---|---|
1 | 730681 | 214.2833 |
2 | 334212 | 57.7037 |
3 | 571362 | 223.1813 |
4 | 907331 | 219.9710 |
5 | 208382 | 55.4499 |
6 | 852863 | 216.6935 |
7 | 494644 | 56.6413 |
8 | 852863 | 216.6935 |
9 | 521061 | 68.0861 |
10 | 907023 | 53.8339 |
11 | 906063 | 67.2746 |
12 | 558053 | 67.3586 |
13 | 832804 | 205.2770 |
14 | 554976 | 208.7227 |
⋮ |
You are now following this blog post
You will see updates in your activity feed.
You may receive emails, depending on your notification preferences.
- Category:
- Data Science
Comments
To leave a comment, please click here to sign in to your MathWorks Account or create a new one.